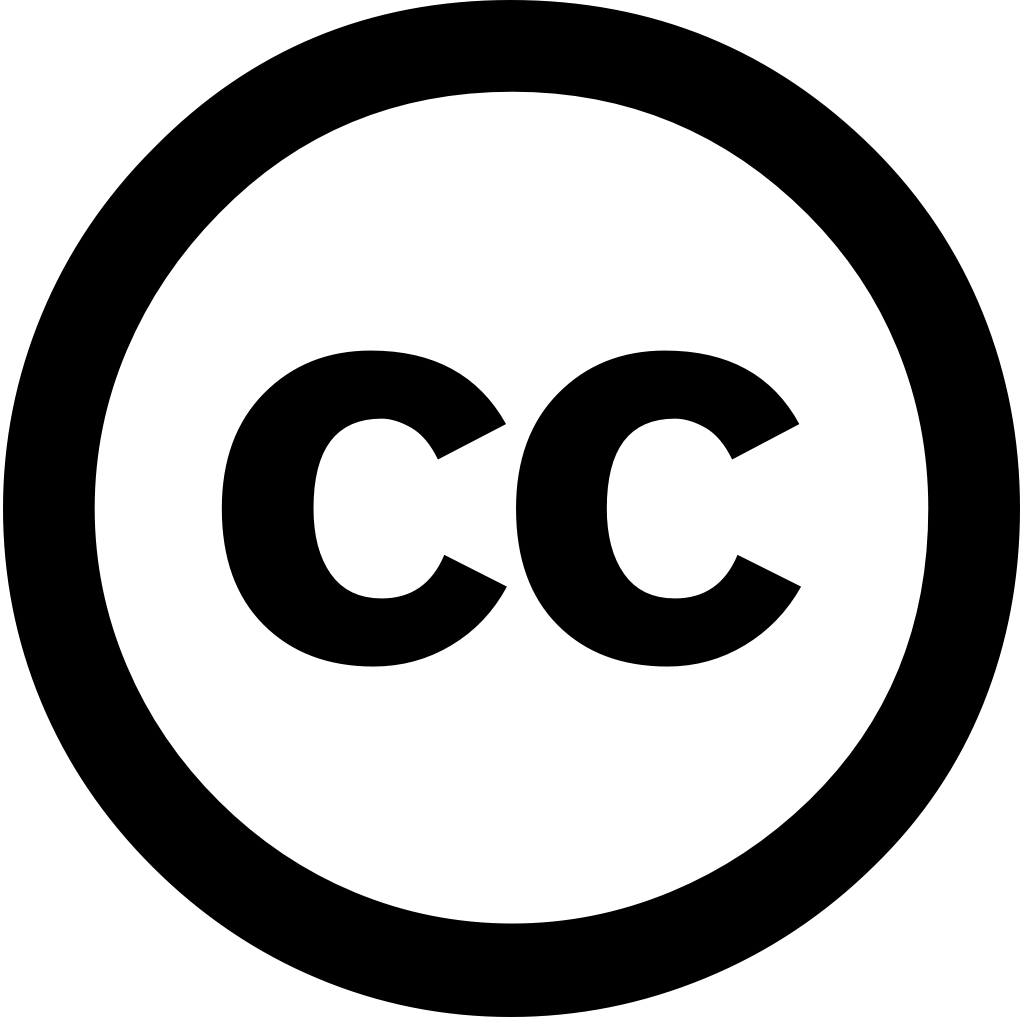
Frontiers in Oncology, Journal Year: 2024, Volume and Issue: 14
Published: Aug. 29, 2024
Introduction
Early
detection
of
pancreatic
cancer
continues
to
be
a
challenge
due
the
difficulty
in
accurately
identifying
specific
signs
or
symptoms
that
might
correlate
with
onset
cancer.
Unlike
breast
colon
prostate
where
screening
tests
are
often
useful
cancerous
development,
there
no
diagnose
cancers.
As
result,
most
cancers
diagnosed
at
an
advanced
stage,
treatment
options,
whether
systemic
therapy,
radiation,
surgical
interventions,
offer
limited
efficacy.
Methods
A
two-stage
weakly
supervised
deep
learning-based
model
has
been
proposed
identify
tumors
using
computed
tomography
(CT)
images
from
Henry
Ford
Health
(HFH)
and
publicly
available
Memorial
Sloan
Kettering
Cancer
Center
(MSKCC)
data
sets.
In
first
nnU-Net
segmentation
was
used
crop
area
location
pancreas,
which
trained
on
MSKCC
repository
281
patient
image
sets
established
tumors.
second
multi-instance
classification
applied
cropped
pancreas
region
segregate
normal
appearing
pancreas.
The
trained,
tested,
validated
obtained
HFH
463
cases
2,882
controls.
Results
learning
model,
architecture,
offers
accuracy
0.907
Language: Английский