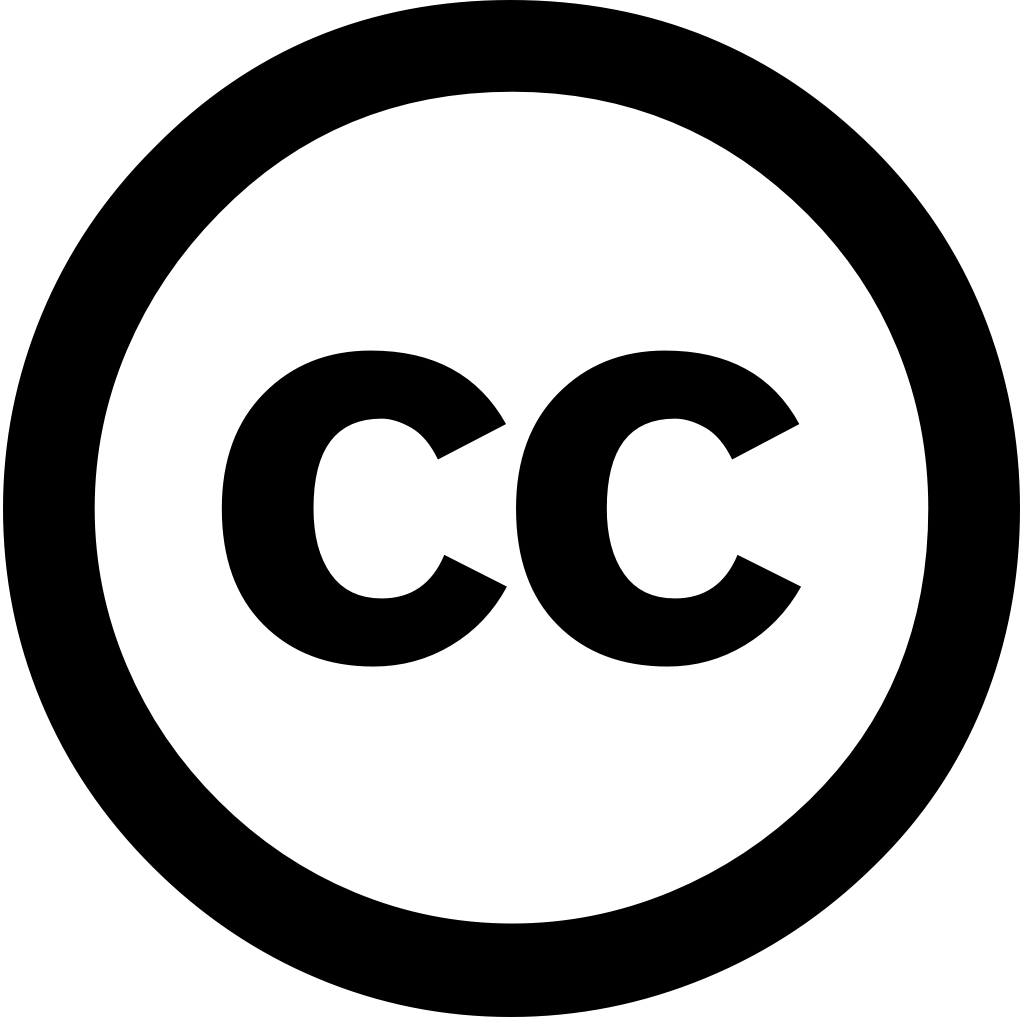
Computerized Medical Imaging and Graphics, Journal Year: 2024, Volume and Issue: 118, P. 102464 - 102464
Published: Nov. 19, 2024
Language: Английский
Computerized Medical Imaging and Graphics, Journal Year: 2024, Volume and Issue: 118, P. 102464 - 102464
Published: Nov. 19, 2024
Language: Английский
Neural Computing and Applications, Journal Year: 2025, Volume and Issue: unknown
Published: Jan. 2, 2025
Language: Английский
Citations
1International Journal of Machine Learning and Cybernetics, Journal Year: 2025, Volume and Issue: unknown
Published: Jan. 28, 2025
Language: Английский
Citations
0Scientific Reports, Journal Year: 2025, Volume and Issue: 15(1)
Published: Feb. 18, 2025
Language: Английский
Citations
0International Journal of Imaging Systems and Technology, Journal Year: 2025, Volume and Issue: 35(2)
Published: March 1, 2025
ABSTRACT Medical image segmentation is a crucial process in medical analysis, with convolutional neural network (CNN)‐based methods achieving notable success recent years. Among these, U‐Net has gained widespread use due to its simple yet effective architecture. However, CNNs still struggle capture global, long‐range semantic information. To address this limitation, we present CS U‐NET, novel method built upon Swin‐U‐Net, which integrates spatial and contextual attention mechanisms. This hybrid approach combines the strengths of both transformers architectures enhance performance. In framework, tokenized patches are processed through transformer‐based U‐shaped encoder‐decoder, enabling learning local global features via skip connections. Our achieves Dice Similarity Coefficient 78.64% 95% Hausdorff distance 21.25 on Synapse multiorgan dataset, outperforming Trans‐U‐Net other state‐of‐the‐art variants by 4% 6%, respectively. The experimental results highlight significant improvements prediction accuracy edge detail preservation provided our approach.
Language: Английский
Citations
0Mathematics, Journal Year: 2024, Volume and Issue: 12(16), P. 2525 - 2525
Published: Aug. 15, 2024
This paper introduces a novel approach to neural networks: Generalized Liquid Neural Network (GLNN) framework. design excels at handling both sequential and non-sequential tasks. By leveraging the Runge Kutta DOPRI method, GLNN enables dynamic simulation of complex systems across diverse fields. Our research demonstrates framework’s capabilities through three key applications. In predicting damped sinusoidal trajectories, LNN outperforms ODE by approximately 46.03% conventional 57.88%. Modelling non-linear RLC circuits shows 20% improvement in precision. Finally, medical diagnosis Optical Coherence Tomography (OCT) image analysis, our achieves an F1 score 0.98, surpassing classical 10%. These advancements signify significant shift, opening new possibilities for networks system modelling healthcare diagnostics. advances field introducing versatile reliable network architecture.
Language: Английский
Citations
2Bioengineering, Journal Year: 2024, Volume and Issue: 11(10), P. 1032 - 1032
Published: Oct. 16, 2024
This paper presents a deep-learning architecture for segmenting retinal fluids in patients with Diabetic Macular Oedema (DME) and Age-related Degeneration (AMD). Accurate segmentation of multiple fluid types is critical diagnosis treatment planning, but existing techniques often struggle precision. We propose an encoder–decoder network inspired by U-Net, processing enhanced OCT images their edge maps. The encoder incorporates Residual Inception modules autoencoder-based multiscale attention mechanism to extract detailed features. Our method shows superior performance across several datasets. On the RETOUCH dataset, achieved F1 Scores 0.82 intraretinal (IRF), 0.93 subretinal (SRF), 0.94 pigment epithelial detachment (PED). model also performed well on OPTIMA DUKE datasets, demonstrating high precision, recall, Scores. significantly enhances accuracy offering valuable tool diagnosing managing diseases. Its integration dual-input processing, attention, advanced highlights its potential improve clinical outcomes advance disease treatment.
Language: Английский
Citations
2npj Digital Medicine, Journal Year: 2024, Volume and Issue: 7(1)
Published: Nov. 5, 2024
Corneal transplantation is the primary treatment for irreversible corneal diseases, but due to limited donor availability, bioengineered equivalents are being developed as a solution, with biocompatibility, structural integrity, and physical function considered key factors. Since conventional evaluation methods may not fully capture complex properties of cornea, there need advanced imaging assessment techniques. In this study, we proposed deep learning-based automatic segmentation method transplanted using optical coherence tomography achieve highly accurate graft integrity biocompatibility. Our provides quantitative individual thickness values, detailed maps, volume measurements equivalents, has been validated through 14 days monitoring. Based on results, it expected have high clinical utility human keratoplasties, including opacity area implanted part extraction, beyond animal studies.
Language: Английский
Citations
2Bioengineering, Journal Year: 2024, Volume and Issue: 11(5), P. 447 - 447
Published: April 30, 2024
Precise medical image segmentation of regions interest (ROIs) is crucial for accurate disease diagnosis and progression assessment. However, acquiring high-quality annotated data at the pixel level poses a significant challenge due to resource-intensive nature this process. This scarcity results in few-shot scenarios, which are highly prevalent clinical applications. To address obstacle, paper introduces Agent-Guided SAM (AGSAM), an innovative approach that transforms Segment Anything Model (SAM) into fully automated method by automating prompt generation. Capitalizing on pre-trained feature extraction decoding capabilities SAM-Med2D, AGSAM circumvents need manual engineering, ensuring adaptability across diverse methods. Furthermore, proposed augmentation convolution module (FACM) enhances model accuracy promoting stable representations. Experimental evaluations demonstrate AGSAM’s consistent superiority over other methods various metrics. These findings highlight efficacy tackling challenges associated with limited while achieving segmentation.
Language: Английский
Citations
1Computerized Medical Imaging and Graphics, Journal Year: 2024, Volume and Issue: 118, P. 102464 - 102464
Published: Nov. 19, 2024
Language: Английский
Citations
0