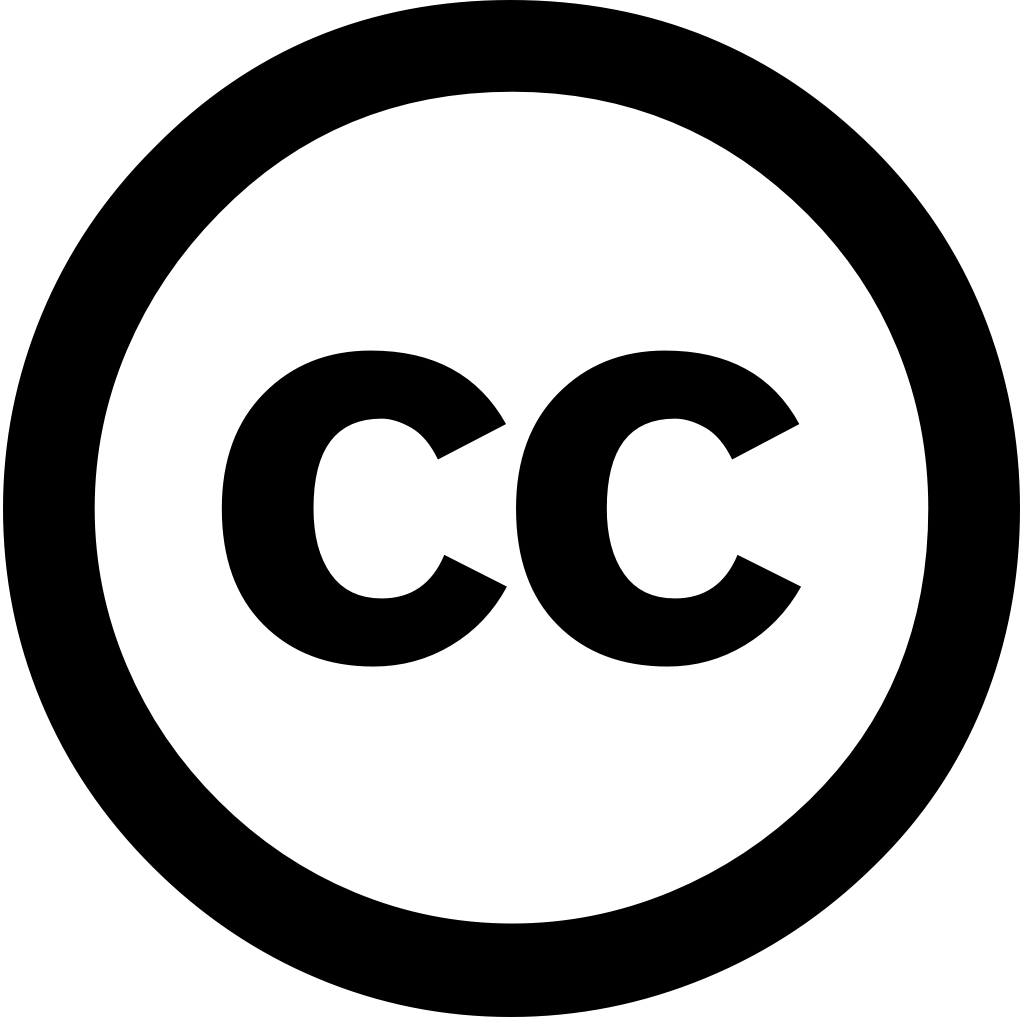
Radiotherapy and Oncology, Journal Year: 2024, Volume and Issue: 196, P. 110325 - 110325
Published: May 10, 2024
Language: Английский
Radiotherapy and Oncology, Journal Year: 2024, Volume and Issue: 196, P. 110325 - 110325
Published: May 10, 2024
Language: Английский
Archives of Computational Methods in Engineering, Journal Year: 2025, Volume and Issue: unknown
Published: Jan. 8, 2025
Abstract Cancer detection has long been a continuous key performer in oncological research. The revolution of artificial intelligence (AI) and its application the field cancer turned out to be more promising recent years. This paper provides detailed review various aspects AI different cancers their staging. role interpreting processing imaging data, accuracy sensitivity detect tumors is examined. images obtained through modalities like MRI, CT, ultrasound etc. are considered this review. Further highlights implementation algorithms 12 types breast cancer, prostate lung as discussed studies. served summarize challenges involved with application. It revealed efficacy detecting region, size, grade cancer. While CT proved ideal for detection, MRI was helpful bestows roadmap fully utilize potential early staging enhance patient survival.
Language: Английский
Citations
1Physica Medica, Journal Year: 2025, Volume and Issue: 131, P. 104914 - 104914
Published: Feb. 11, 2025
Language: Английский
Citations
1Cancers, Journal Year: 2025, Volume and Issue: 17(5), P. 882 - 882
Published: March 4, 2025
According to data from the World Health Organization (WHO), lung cancer is becoming a global epidemic. It particularly high in list of leading causes death not only developed countries, but also worldwide; furthermore, it holds place terms cancer-related mortality. Nevertheless, many breakthroughs have been made last two decades regarding its management, with one most prominent being implementation artificial intelligence (AI) various aspects disease management. We included 473 papers this thorough review, which published during 5-10 years, order describe these breakthroughs. In screening programs, AI capable detecting suspicious nodules different imaging modalities-such as chest X-rays, computed tomography (CT), and positron emission (PET) scans-but discriminating between benign malignant well, success rates comparable or even better than those experienced radiologists. Furthermore, seems be able recognize biomarkers that appear patients who may develop cancer, years before event. Moreover, can assist pathologists cytologists recognizing type tumor, well specific histologic genetic markers play key role treating disease. Finally, treatment field, guide development personalized options for patients, possibly improving their prognosis.
Language: Английский
Citations
1Lung Cancer, Journal Year: 2022, Volume and Issue: 176, P. 4 - 13
Published: Dec. 15, 2022
Language: Английский
Citations
29PLoS ONE, Journal Year: 2023, Volume and Issue: 18(10), P. e0292063 - e0292063
Published: Oct. 5, 2023
Background and purpose In comparison to conventional medical imaging diagnostic modalities, the aim of this overview article is analyze accuracy application Artificial Intelligence (AI) techniques in identification diagnosis malignant tumors adult patients. Data sources The acronym PIRDs was used a comprehensive literature search conducted on PubMed, Cochrane, Scopus, Web Science, LILACS, Embase, Scielo, EBSCOhost, grey through Proquest, Google Scholar, JSTOR for systematic reviews AI as model and/or detection tool any cancer type patients, compared traditional radiographic model. There were no limits publishing status, publication time, or language. For study selection risk bias evaluation, pairs reviewers worked separately. Results total, 382 records retrieved databases, 364 after removing duplicates, 32 satisfied full-text reading criterion, 09 papers considered qualitative synthesis. Although there heterogeneity terms methodological aspects, patient differences, used, studies found that several approaches are promising specificity, sensitivity, tumors. When other machine learning algorithms, Super Vector Machine method performed better diagnosis. Computer-assisted (CAD) has shown aiding detection, when Conclusions with help seems be feasible accurate use different technologies, such CAD systems, deep algorithms radiomic analysis model, although these technologies not capable replace professional radiologist images. limitations regarding generalization all types cancer, tools might aid professionals, serving an auxiliary teaching tool, especially less trained professionals. Therefore, further longitudinal longer follow-up duration required understanding clinical artificial intelligence systems. Trial registration Systematic review registration. Prospero number: CRD42022307403 .
Language: Английский
Citations
20Biomedicines, Journal Year: 2024, Volume and Issue: 12(3), P. 472 - 472
Published: Feb. 20, 2024
The aim of our study was to predict the occurrence distant metastases in non-small-cell lung cancer (NSCLC) patients using machine learning methods and texture analysis 18F-labeled 2-deoxy-d-glucose Positron Emission Tomography/Computed Tomography {[18F]FDG PET/CT} images. In this retrospective single-center study, we evaluated 79 with advanced NSCLC who had undergone [18F]FDG PET/CT scan at diagnosis before any therapy. Patients were divided into two independent training (n = 44) final testing 35) cohorts. Texture features primary tumors lymph node extracted from images LIFEx program. Six applied dataset entire panel features. Dedicated selection used generate different combinations five performance selected determined accuracy, confusion matrix, receiver operating characteristic (ROC) curves, area under curve (AUC). A total 104 78 lesions analyzed cohorts, respectively. support vector (SVM) decision tree showed highest accuracy cohort. Seven obtained introduced models subsequently cohorts SVM tree. AUC method higher than those best combination included shape sphericity, gray level run length matrix_run non-uniformity (GLRLM_RLNU), Total Lesion Glycolysis (TLG), Metabolic Tumor Volume (MTV), compacity. these could an 74.4% 0.63 patients.
Language: Английский
Citations
7Scientific Reports, Journal Year: 2024, Volume and Issue: 14(1)
Published: July 10, 2024
Develop a radiomics nomogram that integrates deep learning, radiomics, and clinical variables to predict epidermal growth factor receptor (EGFR) mutation status in patients with stage I non-small cell lung cancer (NSCLC). We retrospectively included 438 who underwent curative surgery completed driver-gene tests for NSCLC from four academic medical centers. Predictive models were established by extracting analyzing radiomic features intratumoral, peritumoral, habitat regions of CT images identify EGFR NSCLC. Additionally, three learning based on the intratumoral region constructed. A was developed integrating representative signatures, features. Model performance assessed calculating area under receiver operating characteristic (ROC) curve. The demonstrated encouraging discriminating between mutant wild-type, predictive ability superior other single (AUC 0.886, 0.812, 0.790 training, validation, external test sets, respectively). radiomics-based exhibited excellent performance, achieving highest AUC values 0.917, 0.837, 0.809 respectively. Decision curve analysis (DCA) indicated provided higher net benefit than models, offering valuable information treatment.
Language: Английский
Citations
7Indian Journal of Surgical Oncology, Journal Year: 2024, Volume and Issue: 16(1), P. 257 - 278
Published: Sept. 5, 2024
Language: Английский
Citations
7Bioengineering, Journal Year: 2023, Volume and Issue: 10(2), P. 138 - 138
Published: Jan. 20, 2023
The Internet of Things (IoT) has been influential in predicting major diseases current practice. deep learning (DL) technique is vital monitoring and controlling the functioning healthcare system ensuring an effective decision-making process. In this study, we aimed to develop a framework implementing IoT DL identify lung cancer. accurate efficient prediction disease challenging task. proposed model deploys process with multi-layered non-local Bayes (NL Bayes) manage early diagnosis. Medical (IoMT) could be useful determining factors that enable sorting quality values through use sensors image processing techniques. We studied by analyzing its results regard specific attributes such as accuracy, quality, efficiency. overcome problems existing practical computational comparison provided low error rate (2%, 5%) increase number instance values. experimental led us conclude can make predictions based on images high sensitivity better precision compared other results. achieved expected accuracy (81%, 95%), specificity (80%, 98%), 99%). This adequate for real-time health systems cancer
Language: Английский
Citations
16Nature, Journal Year: 2023, Volume and Issue: unknown
Published: Feb. 24, 2023
Language: Английский
Citations
14