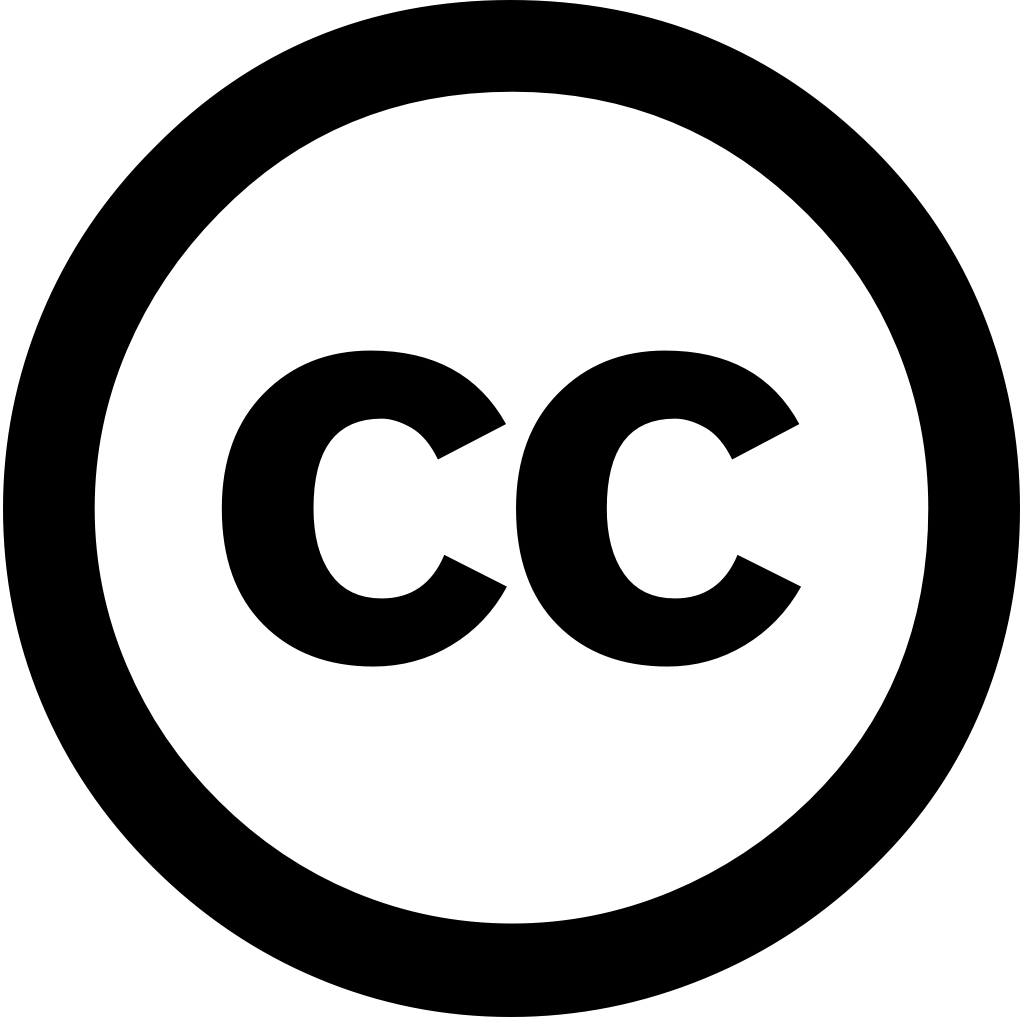
Biomedical Signal Processing and Control, Journal Year: 2024, Volume and Issue: 99, P. 106855 - 106855
Published: Sept. 12, 2024
Language: Английский
Biomedical Signal Processing and Control, Journal Year: 2024, Volume and Issue: 99, P. 106855 - 106855
Published: Sept. 12, 2024
Language: Английский
IEEE Access, Journal Year: 2024, Volume and Issue: 12, P. 67880 - 67888
Published: Jan. 1, 2024
Skin cancer recognition poses a significant challenge in the field of deep learning. While conventional convolutional neural networks have been extensively employed for classifying skin images, their fixed receptive limits ability to capture global features present such images. Conversely, transformer-based models that rely on self-attention can effectively model long-range dependencies, but they come with high computational complexity and exhibit certain limitations local feature induction. To address this issue, paper presents novel network named ECRNet. ECRNet has designed both information, it introduces an explicit vision center accomplish purpose. Moreover, fusion module known as CCPA block. This utilizes coordinate attention channel mechanisms extract image enhance representation evaluate performance ECRNet, extensive experimental comparisons were conducted ISIC2018 dataset. The results demonstrate outperforms baseline model, showing improvements 1.19% accuracy (ACC), 1.96% precision, 4.08% recall, 3.28% F1 score.
Language: Английский
Citations
1Cogent Engineering, Journal Year: 2024, Volume and Issue: 11(1)
Published: Aug. 28, 2024
Skin cancer, a life-threatening disease, necessitates early detection and accurate classification for successful treatment. Misdiagnoses can lead to significant consequences patients, highlighting the critical need improved accuracy. Despite advancements in machine learning (ML) deep (DL) techniques, diagnosis remains challenging due its complex nature. This comprehensive Systematic Literature Review (SLR) aims examine use of ML DL models skin cancer classification, including convolutional neural networks (CNNs), recurrent (RNNs), transfer learning. Model performance is evaluated based on accuracy, sensitivity, specificity, precision. Key findings reveal dominance models, with SVM-PSO emerging as top-performing hybrid model 97.50% Tailored such M-SVM FCN-ResAlexNet, demonstrate high emphasizing importance customization dermatology tasks. Deep networks, ResNet-50, ResNet34, Inception V3, ResNet 152, consistently exhibit strong performance, impact architectural depth. Traditional algorithms like Random Forest, KNN, Naive Bayes face challenges compared models. Furthermore, analysis explores correlations between dataset size revealing varied responses. Temporal trends model-specific analyses uncover outliers, anomalies, influence specific datasets (e.g. imbalanced classes), providing valuable insights future research development. multifaceted nature influenced by factors beyond size, underscores nuanced considerations image classification. Overall, this SLR offer researchers practitioners, serving crucial step towards developing even more reliable tools diagnosis.
Language: Английский
Citations
1Engineering Applications of Artificial Intelligence, Journal Year: 2024, Volume and Issue: 137, P. 109213 - 109213
Published: Aug. 29, 2024
Language: Английский
Citations
1Automatika, Journal Year: 2024, Volume and Issue: 65(4), P. 1458 - 1468
Published: Aug. 29, 2024
Skin cancer has become a serious disease which the potential to scale up if it is not identified earlier. It imperative detect and give treatment skin promptly. Diagnosing manually takes lot of time costly, probability false diagnosis increased due outstanding resemblances among various lesions. Enhancing classification multi-class lesions needs development investigative systems should be automated. Data augmentation with GANs Adaptive Conditional Generative Adversarial Network strategies improves performance. The performance tested using balanced unbalanced datasets. Using proper process data, suggested system attains 94% accuracy for VGG16, 93% ResNet50 94.25% ResNet101. collaboration all such methods further 95%. In summary, novelty work lies in its holistic approach automated lesion classification, incorporating advanced deep learning models, novel data techniques comprehensive evaluation on real-world These contributions collectively advance field computer-aided detection treatment.
Language: Английский
Citations
1Biomedical Signal Processing and Control, Journal Year: 2024, Volume and Issue: 99, P. 106855 - 106855
Published: Sept. 12, 2024
Language: Английский
Citations
1