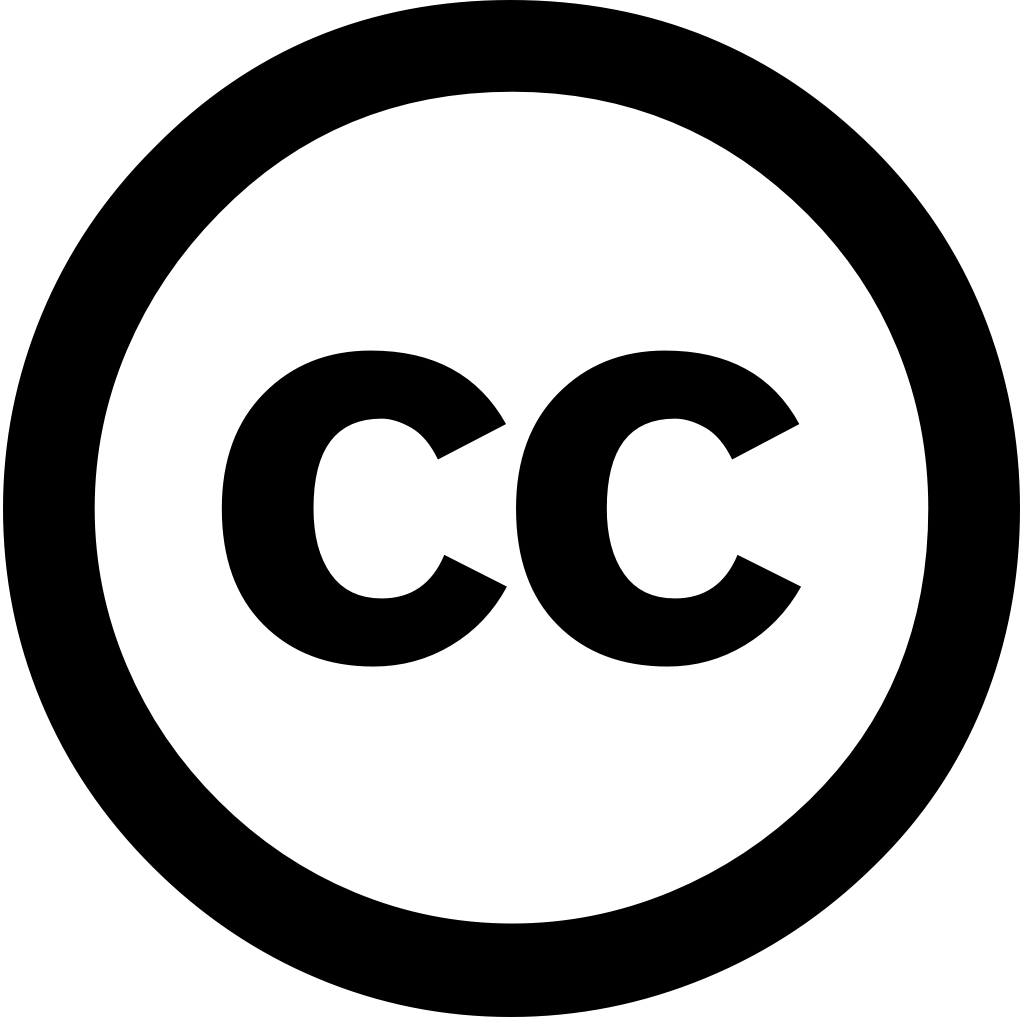
IEEE Access, Journal Year: 2024, Volume and Issue: 12, P. 171368 - 171395
Published: Jan. 1, 2024
Language: Английский
IEEE Access, Journal Year: 2024, Volume and Issue: 12, P. 171368 - 171395
Published: Jan. 1, 2024
Language: Английский
Energies, Journal Year: 2025, Volume and Issue: 18(7), P. 1724 - 1724
Published: March 30, 2025
The integration of renewable energy systems into modern buildings is essential for enhancing efficiency, reducing carbon footprints, and advancing intelligent management. However, optimizing RES operations within building management introduces significant complexity, requiring advanced control strategies. One branch algorithms concerns reinforcement learning, a data-driven strategy capable dynamically managing sources other subsystems under uncertainty real-time constraints. current review systematically examines RL-based strategies applied in BEMS frameworks integrating technologies between 2015 2025, classifying them by algorithmic approach evaluating the role multi-agent hybrid methods improving adaptability occupant comfort. Following thorough explanation rigorous selection process—which targeted most impactful peer-reviewed publications from last decade, paper presents mathematical concepts RL RL, along with detailed summaries summary tables integrated works to facilitate quick reference key findings. For evaluation, outlines different attributes field considering following: methodologies RL; agent types; value-action networks; reward functions; baseline approaches; typologies. Grounded on findings presented evaluation section, offers structured synthesis emerging research trends future directions, identifying strengths limitations
Language: Английский
Citations
1Journal of Building Engineering, Journal Year: 2024, Volume and Issue: unknown, P. 111080 - 111080
Published: Oct. 1, 2024
Language: Английский
Citations
5Energies, Journal Year: 2025, Volume and Issue: 18(6), P. 1526 - 1526
Published: March 19, 2025
The operation of Heating Ventilation and Air Conditioning (HVAC) systems in densely occupied spaces results considerable energy consumption. In the post-pandemic context, stricter indoor air quality standards higher ventilation rates further increase demand. this paper, retrofit a partial recirculation all-air HVAC system serving university lecture room located Southern Italy is analyzed. Multi-Objective Optimization (MOO) Multi-Criteria Decision-Making (MCDM) approaches are used to find optimal design alternatives rank these considering two different decision-makers, i.e., public private stakeholders. Among Pareto solutions obtained from optimization, alternative identified, encompassing three Key Performance Indicators using new robust MCDM approach based on four methods, TOPSIS, VIKOR, WASPAS, MULTIMOORA. show that, era, baseline scenarios for infection reduction that do not involve introduction demand control strategies cause consumption negligible values up 59%. On contrary, involving decrease between 5% 38%. findings offer valuable guidance retrofits education similar buildings, emphasizing potential balance occupant health, efficiency, cost reduction. also highlight significant CO2 reductions minimal impacts thermal comfort, showcasing substantial savings through targeted retrofits.
Language: Английский
Citations
0International Journal of Refrigeration, Journal Year: 2025, Volume and Issue: unknown
Published: May 1, 2025
Language: Английский
Citations
0Infrastructures, Journal Year: 2025, Volume and Issue: 10(5), P. 114 - 114
Published: May 6, 2025
Traffic signal control plays a pivotal role in intelligent transportation systems, directly affecting urban mobility, congestion mitigation, and environmental sustainability. As traffic networks become more dynamic complex, traditional strategies such as fixed-time actuated increasingly fall short addressing real-time variability. In response, adaptive control—powered predominantly by reinforcement learning—has emerged promising data-driven solution for optimizing operations evolving environments. The current review presents comprehensive analysis of high-impact reinforcement-learning-based methods, evaluating their contributions across numerous key dimensions: methodology type, multi-agent architectures, reward design, performance evaluation, baseline comparison, network scale, practical applicability, simulation platforms. Through systematic examination the most influential studies, identifies dominant trends, unresolved challenges, strategic directions future research. findings underscore transformative potential RL enabling intelligent, responsive, sustainable management marking significant shift toward next-generation mobility solutions.
Language: Английский
Citations
0Results in Engineering, Journal Year: 2024, Volume and Issue: unknown, P. 103765 - 103765
Published: Dec. 1, 2024
Language: Английский
Citations
3IEEE Access, Journal Year: 2024, Volume and Issue: 12, P. 171368 - 171395
Published: Jan. 1, 2024
Language: Английский
Citations
1