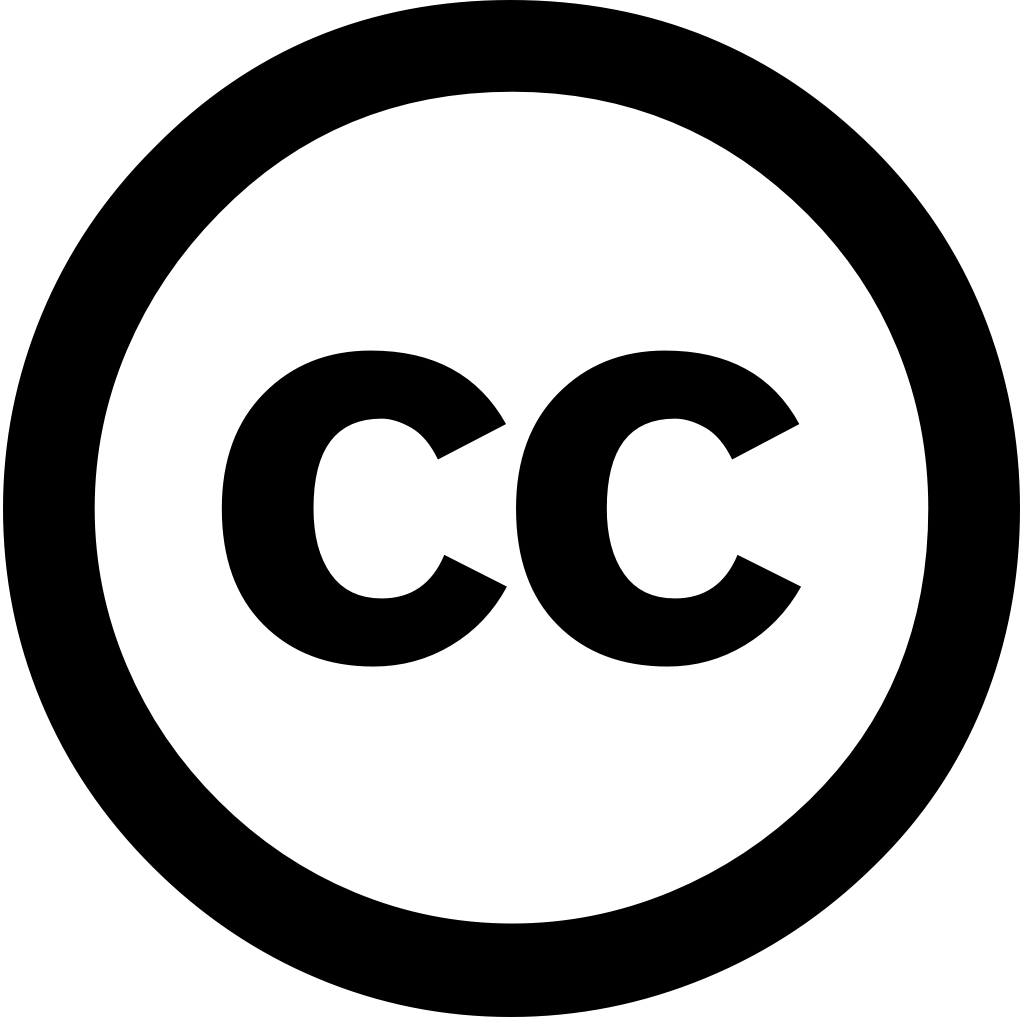
Environmental Pollution and Management, Journal Year: 2024, Volume and Issue: unknown
Published: Nov. 1, 2024
Language: Английский
Environmental Pollution and Management, Journal Year: 2024, Volume and Issue: unknown
Published: Nov. 1, 2024
Language: Английский
Chemical Engineering Journal, Journal Year: 2023, Volume and Issue: 467, P. 143483 - 143483
Published: May 15, 2023
Language: Английский
Citations
65Ecotoxicology and Environmental Safety, Journal Year: 2025, Volume and Issue: 291, P. 117609 - 117609
Published: Feb. 1, 2025
Groundwater pollution, particularly in retired pesticide sites, is a significant environmental concern due to the presence of chlorinated aliphatic hydrocarbons (CAHs) and benzene, toluene, ethylbenzene, xylene (BTEX). These contaminants pose serious risks ecosystems human health. Natural attenuation (NA) has emerged as sustainable solution, with microorganisms playing crucial role pollutant biodegradation. However, interpretation diverse microbial communities relation complex pollutants still challenging, there limited research multi-polluted groundwater. Advanced machine learning (ML) algorithms help identify key indicators for different pollution types (CAHs, BTEX plumes, mixed plumes). The accuracy Area Under Curve (AUC) achieved by Support Vector Machines (SVM) were impressive, values 0.87 0.99, respectively. With assistance model explanation methods, we identified bioindicators which then analyzed using co-occurrence network analysis better understand their potential roles degradation. genera indicate that oxidation co-metabolism predominantly drive dechlorination processes within CAHs group. In group, primary mechanism degradation was observed be anaerobic under sulfate-reducing conditions. CAHs&BTEX groups, indicative suggested occurred iron-reducing conditions reductive existed. Overall, this study establishes framework harnessing power ML alongside based on microbiome data enhance understanding provide robust assessment natural process at sites.
Language: Английский
Citations
2Journal of Cleaner Production, Journal Year: 2024, Volume and Issue: 442, P. 141152 - 141152
Published: Feb. 1, 2024
Language: Английский
Citations
11The Science of The Total Environment, Journal Year: 2024, Volume and Issue: 918, P. 170506 - 170506
Published: Feb. 2, 2024
Language: Английский
Citations
9ACS ES&T Water, Journal Year: 2024, Volume and Issue: 4(4), P. 1904 - 1915
Published: April 2, 2024
Models are increasingly being utilized to improve the understanding and operation of wastewater treatment plants (WWTPs) in face escalating water resource challenges. Abundant operational data provide extensive opportunities for development machine learning (ML) deep (DL) models. However, coupling time lag among features exacerbate black-box nature such models, hindering their application WWTPs. In this study, we construct a DL model using long short-term memory (LSTM) algorithm capable accurately predicting effluent quality full-scale WWTP with finely tuned hyperparameters rationally chosen input features. Comprehensive explanation based on Shapley additive explanations (SHAP) is implemented clarify contributions multivariate series (MTS) inputs predicted results terms feature dimensions. The LSTM models exhibit excellent accuracy (R2 0.96, 0.95, 0.76 MAPE 5.49, 7.17, 13.37%, respectively) chemical oxygen demand (COD), total phosphorus (TP), nitrogen (TN) better than other baseline ML SHAP quantify what most important when they exert influence how impact results. analysis from temporal dimension further explains characteristics process justifies introduction MTS. Compared correlation without engineering, selection method by significantly enhances predictive accuracy. combinations adjusted values, strong interactions significant output identified. This novel attempt both explainability MTS prediction work shows potential applying WWTPs performance.
Language: Английский
Citations
9Water Resources Management, Journal Year: 2023, Volume and Issue: 37(9), P. 3699 - 3714
Published: May 3, 2023
Language: Английский
Citations
18Applied Sciences, Journal Year: 2024, Volume and Issue: 14(9), P. 3675 - 3675
Published: April 25, 2024
The pollution of groundwater and soil by hydrocarbons is a significant growing global problem. Efforts to mitigate minimise risks are often based on modelling. Modelling-based solutions for prediction control play critical role in preserving dwindling water resources facilitating remediation. objectives this article to: (i) provide concise overview the mechanisms that influence migration improve understanding processes affect contamination levels, (ii) compile most commonly used models simulate fate subsurface; (iii) evaluate these terms their functionality, limitations, requirements. aim enable potential users make an informed decision regarding modelling approaches (deterministic, stochastic, hybrid) match expectations with characteristics models. review 11 1D screening models, 18 deterministic 7 stochastic tools, machine learning experiments aimed at hydrocarbon subsurface should solid basis capabilities each method applications.
Language: Английский
Citations
3Groundwater for Sustainable Development, Journal Year: 2024, Volume and Issue: 26, P. 101272 - 101272
Published: July 4, 2024
Language: Английский
Citations
3Chemical Engineering Journal, Journal Year: 2023, Volume and Issue: 478, P. 147266 - 147266
Published: Nov. 10, 2023
Language: Английский
Citations
6Published: April 26, 2024
Water quality monitoring and prediction plays an energetic role in the preservation of water resources, decision-making, environmental management. The freshwater resources have been severely impacted by contamination due to rising industrialization rapid economic growth. Quality Index (WQI) is a approach which utilized determine status ground surface systems from physicochemical bacteriological information. In recent years, various researchers discovered achievable ways for accurate using latest machine learning deep techniques. This survey represents methodologies like Random Forest (RF), Principal Component Analysis (PCA), Naïve Bayes, Artificial Neural Network (ANN), Recurrent (RNN), Long-Short Term Memory (LSTM), so on are used analysis. RNN LSTM give more results compared other survey, concludes that difficult task factors involved prediction.
Language: Английский
Citations
1