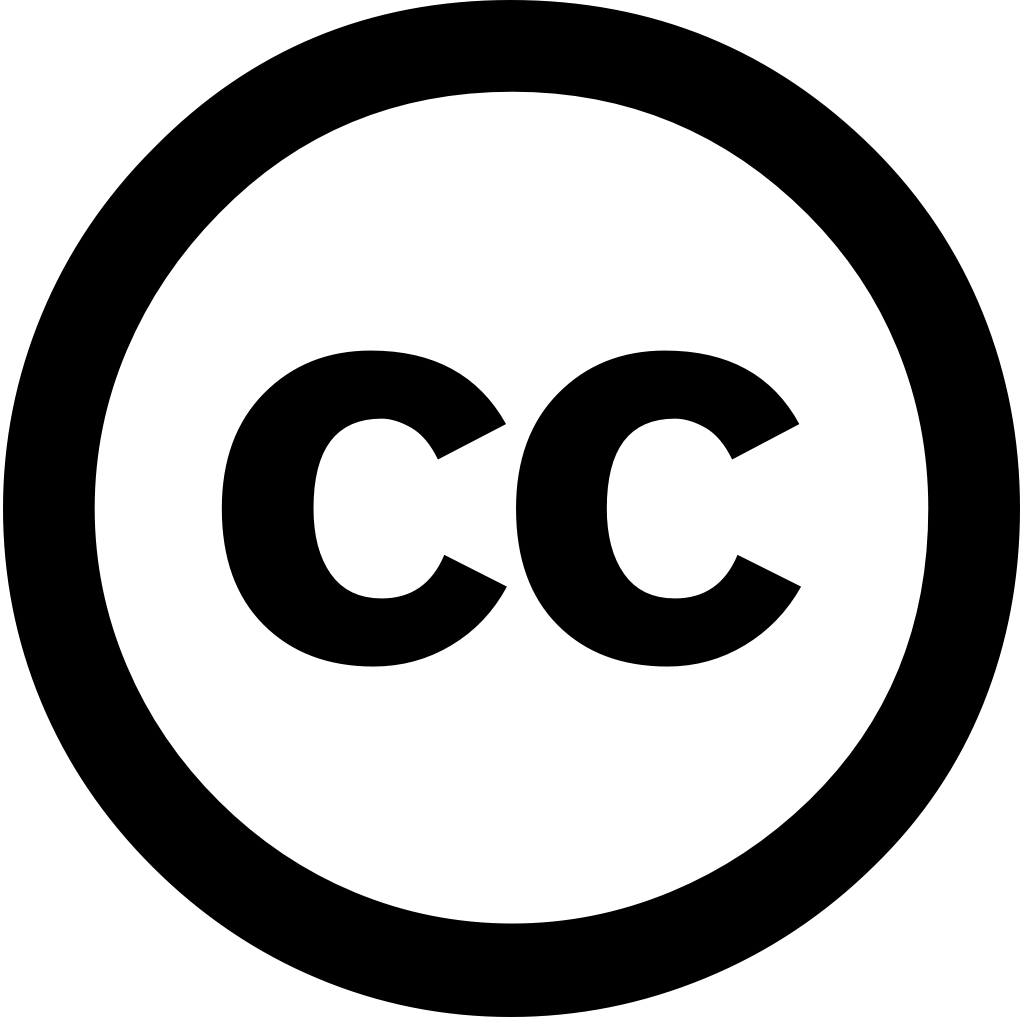
Results in Engineering, Journal Year: 2025, Volume and Issue: unknown, P. 105182 - 105182
Published: May 1, 2025
Language: Английский
Results in Engineering, Journal Year: 2025, Volume and Issue: unknown, P. 105182 - 105182
Published: May 1, 2025
Language: Английский
Journal of Water Process Engineering, Journal Year: 2023, Volume and Issue: 54, P. 104041 - 104041
Published: July 19, 2023
Language: Английский
Citations
44Sensors, Journal Year: 2024, Volume and Issue: 24(11), P. 3650 - 3650
Published: June 5, 2024
The use of low-cost environmental sensors has gained significant attention due to their affordability and potential intensify monitoring networks. These enable real-time various parameters, which can help identify pollution hotspots inform targeted mitigation strategies. Low-cost also facilitate citizen science projects, providing more localized granular data, making accessible communities. However, the accuracy reliability data generated by these be a concern, particularly without proper calibration. Calibration is challenging for variability in sensing materials, transducer designs, conditions. Therefore, standardized calibration protocols are necessary ensure sensor data. This review article addresses four critical questions related sensors. Firstly, it discusses why increasingly being used as an alternative high-cost In addition, self-calibration techniques how they outperform traditional techniques. Secondly, highlights importance selectivity sensitivity generating accurate Thirdly, examines impact functions on improved accuracies. Lastly, approaches that adopted improve sensors, such incorporating advanced analysis enhancing material design. reference-grade validation conclusion, have revolutionize monitoring, areas where methods not feasible. successful implementation. innovative enhance design
Language: Английский
Citations
17Water, Journal Year: 2025, Volume and Issue: 17(2), P. 170 - 170
Published: Jan. 10, 2025
Artificial intelligence (AI) uses highly powerful computers to mimic human intelligent behavior; it is a major research hotspot in science and technology, with an increasing number of applications wider range fields, including complex process supervision control. Wastewater treatment example involving many uncertainties external factors achieve final product specific requisites (effluents prescribed quality). Reducing energy consumption, greenhouse gas emissions, resources recovery are additional requirements these facilities’ operation. AI could extend the purpose expected results previously adopted tools present operational approaches by leveraging superior simulation, prediction, control, adaptation capabilities. This paper reviews current wastewater field discusses achievements potentials. So far, almost all sector involve predictive studies, often at small scale or limited data use. Frontline aimed creation AI-supported digital twins real systems being conducted, few encouraging but still applications. aims identifying discussing key barriers adoption field, which include laborious instrumentation maintenance, lack expertise design software, instability control loops, insufficient incentives for resource efficiency achievement.
Language: Английский
Citations
2International Journal of Environmental Research and Public Health, Journal Year: 2022, Volume and Issue: 19(21), P. 14080 - 14080
Published: Oct. 28, 2022
Nowadays, water pollution has become a global issue affecting most countries in the world. Water quality should be monitored to alert authorities on pollution, so that action can taken quickly. The objective of review is study various conventional and modern methods monitoring identify strengths weaknesses methods. include Internet Things (IoT), virtual sensing, cyber-physical system (CPS), optical techniques. In this review, systems process control several countries, such as New Zealand, China, Serbia, Bangladesh, Malaysia, India, are discussed. Conventional compared terms parameters, complexity, reliability. Recent techniques also reviewed any loopholes We found CPS suitable for due good combination physical computational algorithms. Its embedded sensors, processors, actuators designed detect interact with environments. believe costly complex, whereas expensive but simpler real-time detection. Traditional approaches more time-consuming high maintenance laboratory facilities, involve chemical materials, inefficient on-site applications. Apart from that, previous have issues achieving reliable measurement parameters real time. There still limitations instruments detecting pollutants producing valuable information quality. Thus, important order compare improve current assessments reliability cost-effectiveness.
Language: Английский
Citations
67Water Resources Research, Journal Year: 2022, Volume and Issue: 58(11)
Published: Nov. 1, 2022
Abstract Reliable characterization of subsurface structures is essential for earth sciences and related applications. Data assimilation‐based identification frameworks can reasonably estimate using available lithological (e.g., borehole core, well log) dynamic hydraulic head, solute concentration) observations. However, a reasonable selection the observation type frequency accurate structure identification. To achieve this, we extended recently developed stage‐wise stochastic deep learning inversion framework by coupling it with non‐isothermal flow transport simulations. With framework, worth three common observations (hydraulic concentration, temperature) are compared under different noise frequency. The combines emerging deep‐learning (DL)‐based traditional approaches. This combination makes possible to simultaneously compare ability these two methods assimilate data. Our results show that including at least one strongly improves identifiability reduces uncertainty. DL‐based able identify more accurately than same scenarios. Assimilation certain types could reduce prediction error responses, but not necessarily other uncorrelated responses. Observation data affected High increases uncertainty estimation accuracy. higher significantly improve temporal information compensate negative impacts high noise.
Language: Английский
Citations
65Hydrological Processes, Journal Year: 2022, Volume and Issue: 36(4)
Published: March 29, 2022
Abstract The global decline of water quality in rivers and streams has resulted a pressing need to design new watershed management strategies. Water can be affected by multiple stressors including population growth, land use change, warming, extreme events, with repercussions on human ecosystem health. A scientific understanding factors affecting riverine predictions at local regional scales, sub‐daily decadal timescales are needed for optimal watersheds river basins. Here, we discuss how machine learning (ML) enable development more accurate, computationally tractable, scalable models analysis quality. We review relevant state‐of‐the art applications ML opportunities improve the emerging computational mathematical methods model selection, hyperparameter optimization, incorporating process knowledge into models, improving explainablity, uncertainty quantification, model‐data integration. then present considerations using address problems given their scale complexity, available data resources, stakeholder needs. When combined decades understanding, interdisciplinary advances knowledge‐guided ML, information theory, integration, analytics help fundamental science questions decision‐relevant
Language: Английский
Citations
54Future Internet, Journal Year: 2022, Volume and Issue: 14(9), P. 259 - 259
Published: Aug. 30, 2022
With the effects of climate change such as increasing heat, higher rainfall, and more recurrent extreme weather events including storms floods, a unique approach to studying climatic elements on groundwater level variations is required. These approaches will help people make better decisions. Researchers stakeholders can attain these goals if they become familiar with current machine learning mathematical model predicting changes. However, descriptions for forecasting changes are lacking. This study picked 117 papers from Scopus scholarly database address this knowledge gap. In systematic review, publications were examined using quantitative qualitative approaches, Preferred Reporting Items Systematic Reviews Meta-Analyses (PRISMA) was chosen reporting format. Machine techniques have made significant contributions changes, according study. domain skewed because has been popular in recent years, random forest (RF) methods dominating, followed by support vector (SVM) artificial neural network (ANN). ensembles also found aspects computational complexity, performance training times. Furthermore, compared techniques, achieve accuracies, our research. As result, it advised that academics employ new while considering
Language: Английский
Citations
52Sensors, Journal Year: 2023, Volume and Issue: 23(8), P. 3919 - 3919
Published: April 12, 2023
Water is a vital source for life and natural environments. This the reason why water sources should be constantly monitored in order to detect any pollutants that might jeopardize quality of water. paper presents low-cost internet-of-things system capable measuring reporting different sources. It comprises following components: Arduino UNO board, Bluetooth module BT04, temperature sensor DS18B20, pH sensor-SEN0161, TDS sensor-SEN0244, turbidity sensor-SKU SEN0189. The will controlled managed from mobile application, which monitor actual status We propose evaluate five rural settlement. results show most we have are proper consumption, with single exception where values not within limits, as they outperform maximum accepted value 500 ppm.
Language: Английский
Citations
27Sensors, Journal Year: 2024, Volume and Issue: 24(9), P. 2871 - 2871
Published: April 30, 2024
Traditional laboratory-based water quality monitoring and testing approaches are soon to be outdated, mainly because of the need for real-time feedback immediate responses emergencies. The more recent wireless sensor network (WSN)-based techniques evolving alleviate problems monitoring, coverage, energy management, among others. inclusion Internet Things (IoT) in WSN can further lead their improvement delivering, real time, effective efficient water-monitoring systems, reaping from benefits IoT systems. However, they still suffer inability deliver accurate data, a lack reconfigurability, deployed ad hoc harsh environments, limited acceptability within industry. Electronic sensors required them effectively incorporated into water-quality-monitoring system. Very few electronic exist parameter measurement. This necessitates incorporation artificial intelligence (AI) sensory smart systems indicators without actual by relating with available data. approach is its infancy not yet accepted nor standardized work presents framework featuring an intelligent system uses AI sensors, as design lagging behind In particular, machine learning algorithms used predict E. coli concentrations water. Six different models (ridge regression, random forest regressor, stochastic gradient boosting, support vector machine, k-nearest neighbors, AdaBoost regressor) on sourced dataset. From results, best-performing model average during was regressor (a MAE¯ 14.37 counts/100 mL), worst-performing boosting 42.27 mL). development application such trivial. set (Set A) contained pH, conductivity, chloride, turbidity, nitrates, chlorophyll.
Language: Английский
Citations
10Annual Reviews in Control, Journal Year: 2023, Volume and Issue: 55, P. 420 - 441
Published: Jan. 1, 2023
Language: Английский
Citations
18