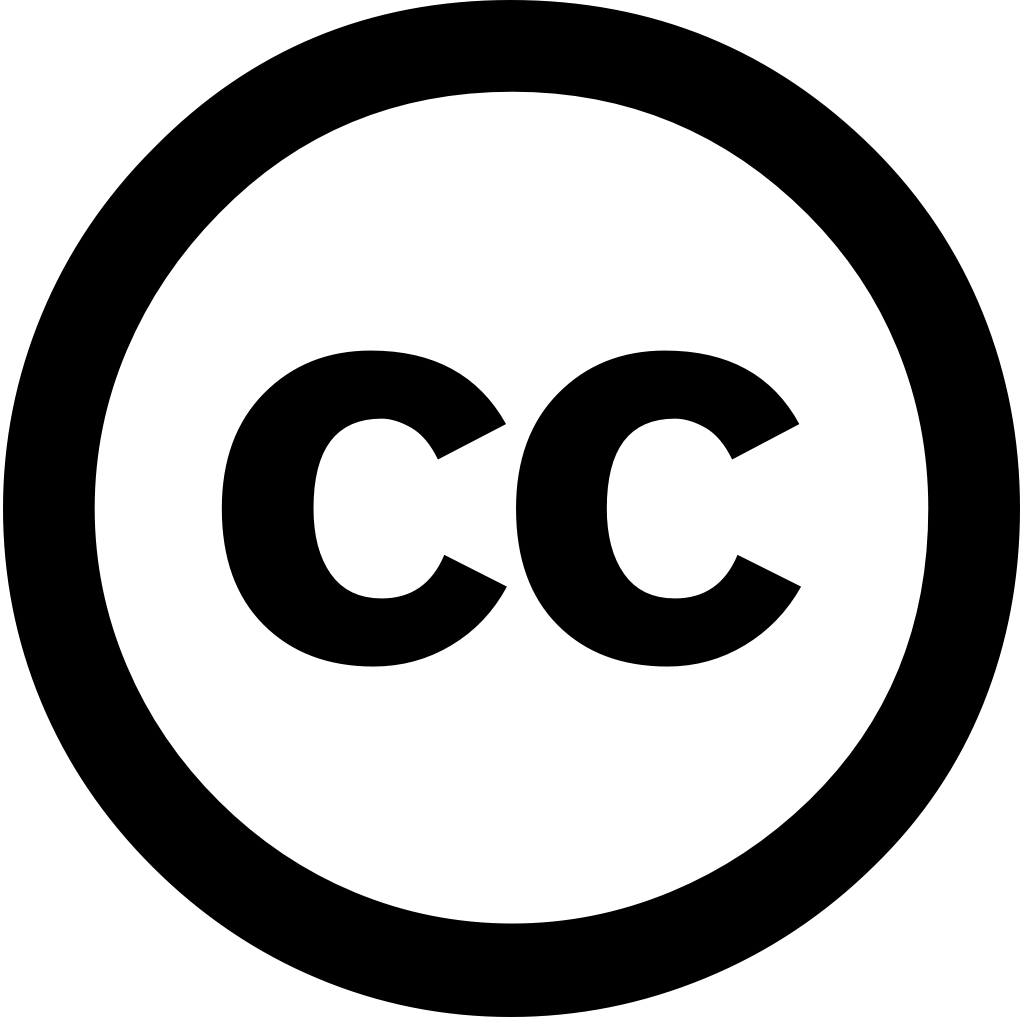
Brain Research, Journal Year: 2024, Volume and Issue: unknown, P. 149423 - 149423
Published: Dec. 1, 2024
Language: Английский
Brain Research, Journal Year: 2024, Volume and Issue: unknown, P. 149423 - 149423
Published: Dec. 1, 2024
Language: Английский
PLoS ONE, Journal Year: 2025, Volume and Issue: 20(4), P. e0314447 - e0314447
Published: April 17, 2025
The functional near-infrared spectroscopy-based brain-computer interface (fNIRS-BCI) systems recognize patterns in brain signals and generate control commands, thereby enabling individuals with motor disabilities to regain autonomy. In this study hand gripping data is acquired using fNIRS neuroimaging system, preprocessing performed nirsLAB features extraction deep learning (DL) Algorithms. For feature classification stack fft methods are proposed. Convolutional neural networks (CNN), long short-term memory (LSTM), bidirectional long-short-term (Bi-LSTM) employed extract features. method classifies these a model the enhances by applying fast Fourier transformation which followed model. proposed applied from twenty participants engaged two-class hand-gripping activity. performance of compared conventional CNN, LSTM, Bi-LSTM algorithms one another. yield 90.11% 87.00% accuracies respectively, significantly higher than those achieved CNN (85.16%), LSTM (79.46%), (81.88%) algorithms. results show that can be effectively used for two three-class problems fNIRS-BCI applications.
Language: Английский
Citations
0Brain Research, Journal Year: 2024, Volume and Issue: unknown, P. 149423 - 149423
Published: Dec. 1, 2024
Language: Английский
Citations
1