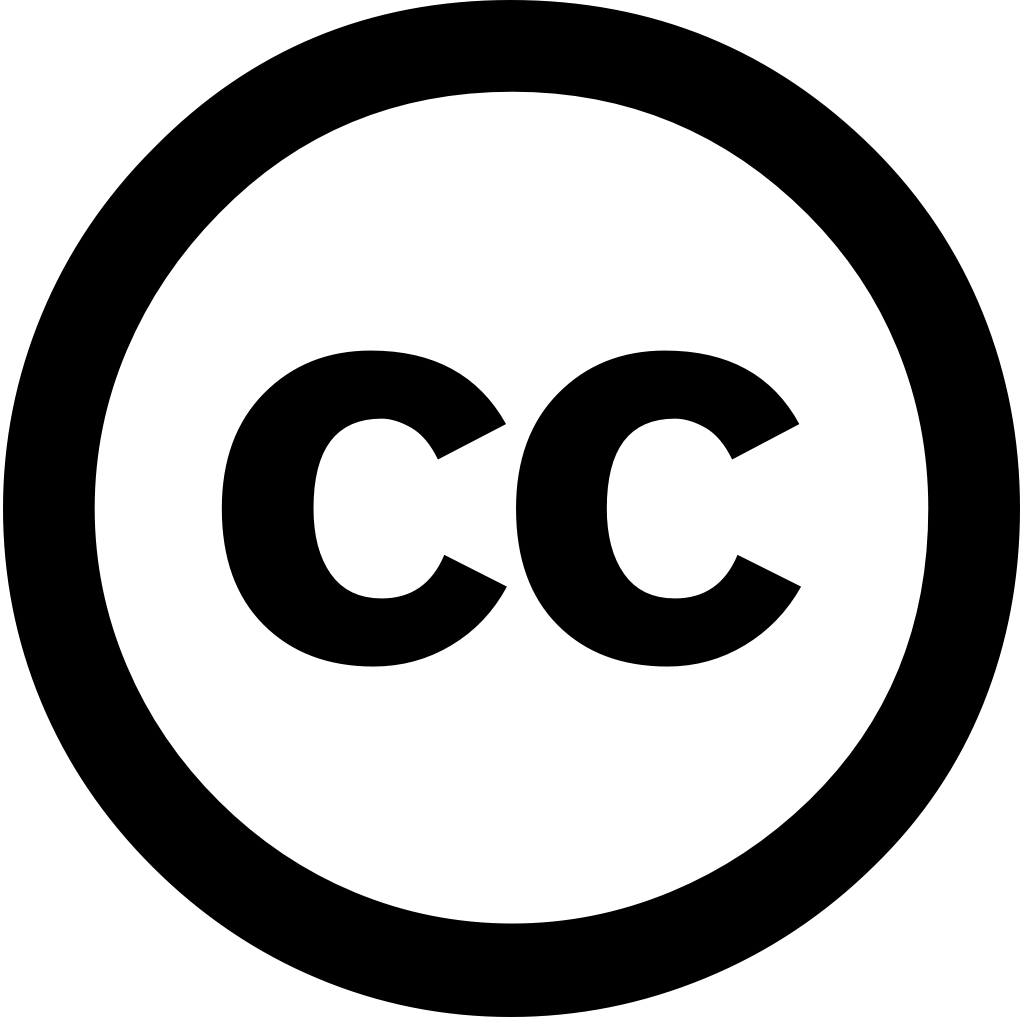
Applied Energy, Journal Year: 2022, Volume and Issue: 328, P. 120113 - 120113
Published: Nov. 1, 2022
Language: Английский
Applied Energy, Journal Year: 2022, Volume and Issue: 328, P. 120113 - 120113
Published: Nov. 1, 2022
Language: Английский
Energy Conversion and Management, Journal Year: 2022, Volume and Issue: 255, P. 115340 - 115340
Published: Feb. 12, 2022
Language: Английский
Citations
54IEEE Transactions on Smart Grid, Journal Year: 2022, Volume and Issue: 14(2), P. 1541 - 1554
Published: Feb. 7, 2022
Local electricity markets (LEM) have recently attracted great interest as an effective solution to the challenging problem of distributed energy resources' (DER) management. However, LEM designs combining market functions local trading and flexibility services (FS) provision wider system operators not sufficient attention. In context addressing this research gap, paper firstly provides a new model-based system-centric formulation for coordination such LEM, which theoretical optimality benchmark. Compared previous formulations, it considers time-coupling operating characteristics flexible DERs, optimizes two simultaneously. Furthermore, explores very first time model-free prosumer-centric approach in order address practical limitations approaches. This is achieved through multi-agent deep reinforcement learning method combines beneficial properties multi-actor-attention-critic prioritized experience replay Case studies on real-world, large-scale setting validate that proposed design successfully encapsulates economic benefits both FS functions, demonstrate outperforms methods.
Language: Английский
Citations
51Energies, Journal Year: 2022, Volume and Issue: 16(1), P. 224 - 224
Published: Dec. 25, 2022
Accurate fault location is challenging due to the distribution network’s various branches, complicated topology, and increasing penetration of distributed energy resources (DERs). The diagnostics for power system faults are based on localization, isolation, smart restoration. Adaptive multi-agent systems (MAS) can improve reliability, speed, selectivity, robustness protection. This paper proposes a MAS-based adaptive protection mechanism in grid applications. study developed novel intelligent-based prevention mitigation technique against electrical cyber-attacks. Simulation studies performed platform constructed by interconnecting Kenitra city MATLAB/SIMULINK implemented JADE platform. simulation results demonstrate effectiveness proposed technique.
Language: Английский
Citations
49Journal of Modern Power Systems and Clean Energy, Journal Year: 2022, Volume and Issue: 10(3), P. 743 - 754
Published: Jan. 1, 2022
This paper develops deep reinforcement learning (DRL) algorithms for optimizing the operation of home energy system which consists photovoltaic (PV) panels, battery storage system, and household appliances. Model-free DRL can efficiently handle difficulty modeling uncertainty PV generation. However, discrete-continuous hybrid action space considered challenges existing either discrete actions or continuous actions. Thus, a mixed (MDRL) algorithm is proposed, integrates Q-learning (DQL) deterministic policy gradient (DDPG) algorithm. The DQL deals with actions, while DDPG handles MDRL learns optimal strategy by trial-and-error interactions environment. unsafe violate constraints, give rise to great cost. To such problem, safe-MDRL further proposed. Simulation studies demonstrate that proposed challenge from management. reduces cost maintaining human thermal comfort comparing benchmark on test dataset. Moreover, greatly loss in stage
Language: Английский
Citations
48Applied Energy, Journal Year: 2022, Volume and Issue: 328, P. 120113 - 120113
Published: Nov. 1, 2022
Language: Английский
Citations
48