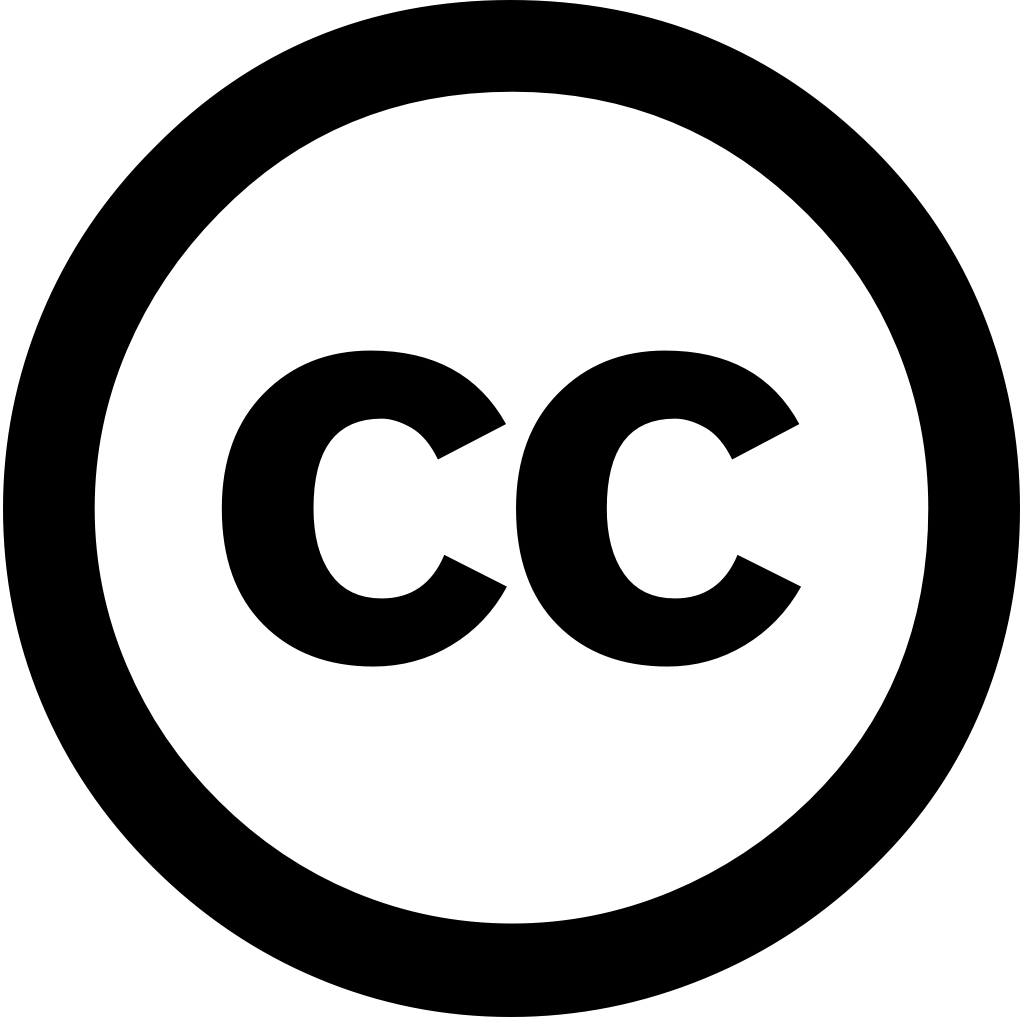
Fuel Processing Technology, Journal Year: 2024, Volume and Issue: 267, P. 108176 - 108176
Published: Dec. 24, 2024
Language: Английский
Fuel Processing Technology, Journal Year: 2024, Volume and Issue: 267, P. 108176 - 108176
Published: Dec. 24, 2024
Language: Английский
Journal of Hazardous Materials, Journal Year: 2024, Volume and Issue: 474, P. 134865 - 134865
Published: June 12, 2024
Language: Английский
Citations
18Marine Pollution Bulletin, Journal Year: 2025, Volume and Issue: 212, P. 117529 - 117529
Published: Jan. 4, 2025
Language: Английский
Citations
1Engineering Applications of Artificial Intelligence, Journal Year: 2025, Volume and Issue: 144, P. 110132 - 110132
Published: Jan. 31, 2025
Language: Английский
Citations
1Marine Pollution Bulletin, Journal Year: 2025, Volume and Issue: 213, P. 117665 - 117665
Published: Feb. 17, 2025
Language: Английский
Citations
0Published: Feb. 21, 2025
Emerging pollutants such as pharmaceuticals, industrial chemicals, heavy metals, and microplastics are a growing ecological risk affecting water soil resources. Another challenge in current wastewater treatments includes tracking treating these pollutants, which can be costly. As concern, emerging do not have lower limit levels detrimental to aquatic resources minuscule amounts. Thus, the assessment of multiple community-based sources surface groundwater is prioritized area study for resource management. It provides basis health management arising diseases cancer dengue caused by unsafe sources. Accordingly, utilizing artificial intelligence, wide-range data-driven insights synthesized assist propose solution pathways without need exhaustive experimentation. This systematic review examines intelligence-assisted modelling notably machine learning deep models, with proximity dependence correlated synergistic effects both humans life. underscores increasing accumulation their toxicological on community how utilized addressing research gaps related treatment methods pollutants.
Language: Английский
Citations
0Trends in Food Science & Technology, Journal Year: 2025, Volume and Issue: unknown, P. 104964 - 104964
Published: March 1, 2025
Language: Английский
Citations
0Water Air & Soil Pollution, Journal Year: 2025, Volume and Issue: 236(5)
Published: April 9, 2025
Language: Английский
Citations
0IEEE Access, Journal Year: 2024, Volume and Issue: 12, P. 134852 - 134861
Published: Jan. 1, 2024
Microplastics from fiber shredding are recognized by the scientific community as one of main sources microplastic water pollution. Therefore, there is a compelling need for techniques capable accurately identifying shredded microplastics in water. The recently released Holography Micro-Plastic Dataset, obtained through use digital holography microscope techniques, offers opportunity to test capability deep neural networks distinguish between and other debris on standard benchmark. promising results initial batch experiments can be further improved employing combined approach involving different image mapping leveraging recent state-of-the-art learning models. Within this framework, we analyze various fusion schemes merge paired dataset images (amplitude phase grayscale images) into single three-channel picture. We demonstrate that our proposed yields increased accuracy compared both single-image data processing techniques. Finally, performance method enhanced utilizing DenseNet model backbone learning-based classification.
Language: Английский
Citations
3Emerging contaminants and associated treatment technologies, Journal Year: 2024, Volume and Issue: unknown, P. 553 - 579
Published: Jan. 1, 2024
Language: Английский
Citations
3Sensors, Journal Year: 2024, Volume and Issue: 24(22), P. 7189 - 7189
Published: Nov. 9, 2024
This study focuses on the classification of six different macrofungi species using advanced deep learning techniques. Fungi species, such as Amanita pantherina, Boletus edulis, Cantharellus cibarius, Lactarius deliciosus, Pleurotus ostreatus and Tricholoma terreum were chosen based their ecological importance distinct morphological characteristics. The research employed 5 machine techniques 12 models, including DenseNet121, MobileNetV2, ConvNeXt, EfficientNet, swin transformers, to evaluate performance in identifying fungi from images. DenseNet121 model demonstrated highest accuracy (92%) AUC score (95%), making it most effective distinguishing between species. also revealed that transformer-based particularly transformer, less effective, suggesting room for improvement application this task. Further advancements could be achieved by expanding datasets, incorporating additional data types biochemical, electron microscopy, RNA/DNA sequences, ensemble methods enhance performance. findings contribute valuable insights into both use biodiversity conservation
Language: Английский
Citations
3