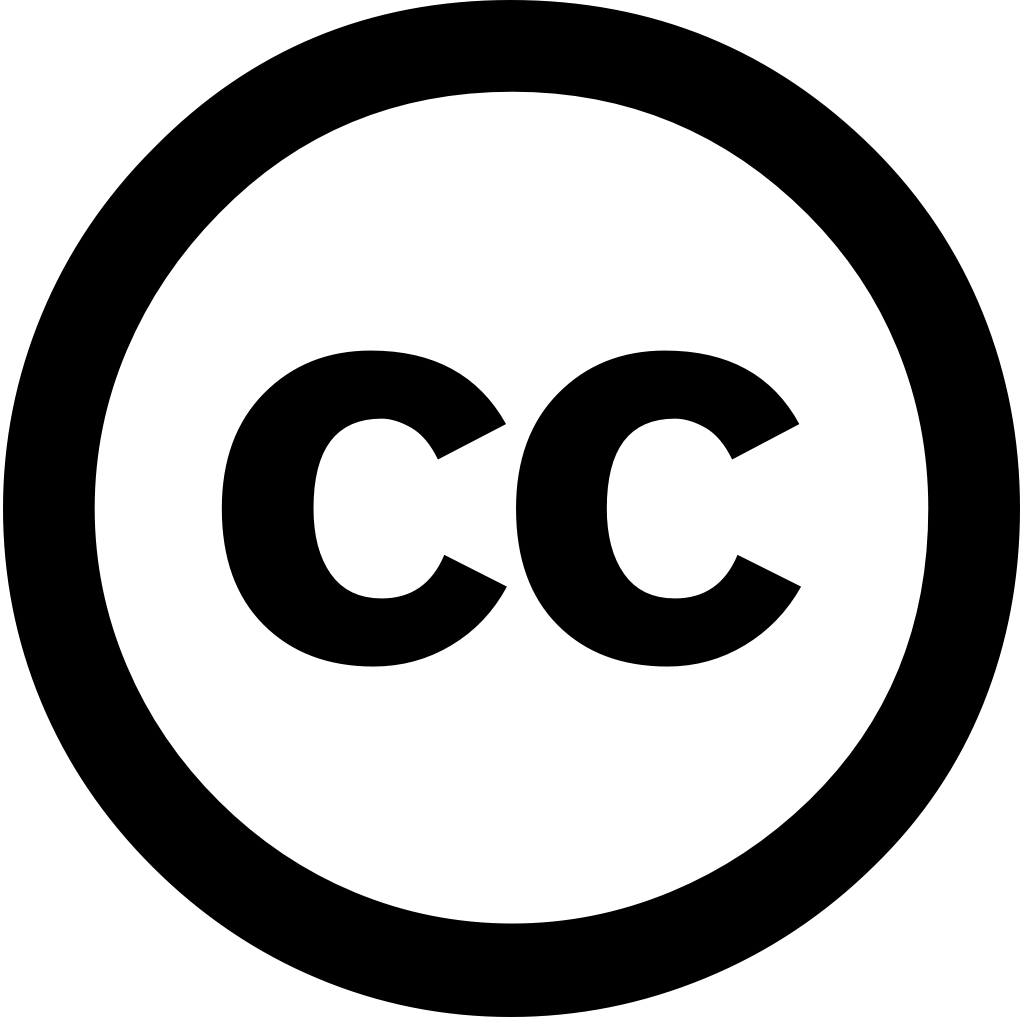
Journal of Hazardous Materials Advances, Journal Year: 2024, Volume and Issue: unknown, P. 100576 - 100576
Published: Dec. 1, 2024
Language: Английский
Journal of Hazardous Materials Advances, Journal Year: 2024, Volume and Issue: unknown, P. 100576 - 100576
Published: Dec. 1, 2024
Language: Английский
Journal of Hazardous Materials Advances, Journal Year: 2025, Volume and Issue: 17, P. 100604 - 100604
Published: Jan. 15, 2025
Language: Английский
Citations
1Smart Agricultural Technology, Journal Year: 2025, Volume and Issue: unknown, P. 100790 - 100790
Published: Jan. 1, 2025
Language: Английский
Citations
1Infrastructures, Journal Year: 2024, Volume and Issue: 9(10), P. 181 - 181
Published: Oct. 9, 2024
This paper explores advanced machine learning approaches to enhance the prediction accuracy of compressive strength (CoS) in geopolymer composites (GePC). Geopolymers, as sustainable alternatives Ordinary Portland Cement (OPC), offer significant environmental benefits by utilizing industrial by-products such fly ash and ground granulated blast furnace slag (GGBS). The accurate their is crucial for optimizing mix design reducing experimental efforts. We present a comparative analysis two hybrid models, Harris Hawks Optimization with Random Forest (HHO-RF) Sine Cosine Algorithm (SCA-RF), against traditional regression methods classical models like Extreme Learning Machine (ELM), General Regression Neural Network (GRNN), Radial Basis Function (RBF). Using comprehensive dataset derived from various scientific publications, we focus on key input variables including fine aggregate, GGBS, ash, sodium hydroxide (NaOH) molarity, others. Our results indicate that SCA-RF model achieved superior performance root mean square error (RMSE) 1.562 coefficient determination (R2) 0.987, compared HHO-RF model, which obtained an RMSE 1.742 R2 0.982. Both significantly outperformed methods, demonstrating higher reliability predicting GePC. research underscores potential advancing construction materials through precise predictive modeling, paving way more environmentally friendly efficient practices.
Language: Английский
Citations
7Journal of environmental chemical engineering, Journal Year: 2024, Volume and Issue: 12(6), P. 114330 - 114330
Published: Oct. 6, 2024
Language: Английский
Citations
4Ecological Informatics, Journal Year: 2024, Volume and Issue: unknown, P. 102982 - 102982
Published: Dec. 1, 2024
Language: Английский
Citations
4The European Physical Journal Plus, Journal Year: 2025, Volume and Issue: 140(3)
Published: March 5, 2025
Language: Английский
Citations
0Environmental Technology & Innovation, Journal Year: 2025, Volume and Issue: unknown, P. 104154 - 104154
Published: March 1, 2025
Language: Английский
Citations
0Rock Mechanics and Rock Engineering, Journal Year: 2025, Volume and Issue: unknown
Published: April 18, 2025
Language: Английский
Citations
0Journal of environmental chemical engineering, Journal Year: 2024, Volume and Issue: 12(5), P. 113328 - 113328
Published: June 14, 2024
Language: Английский
Citations
3Chemosphere, Journal Year: 2024, Volume and Issue: 363, P. 142697 - 142697
Published: June 24, 2024
Language: Английский
Citations
3