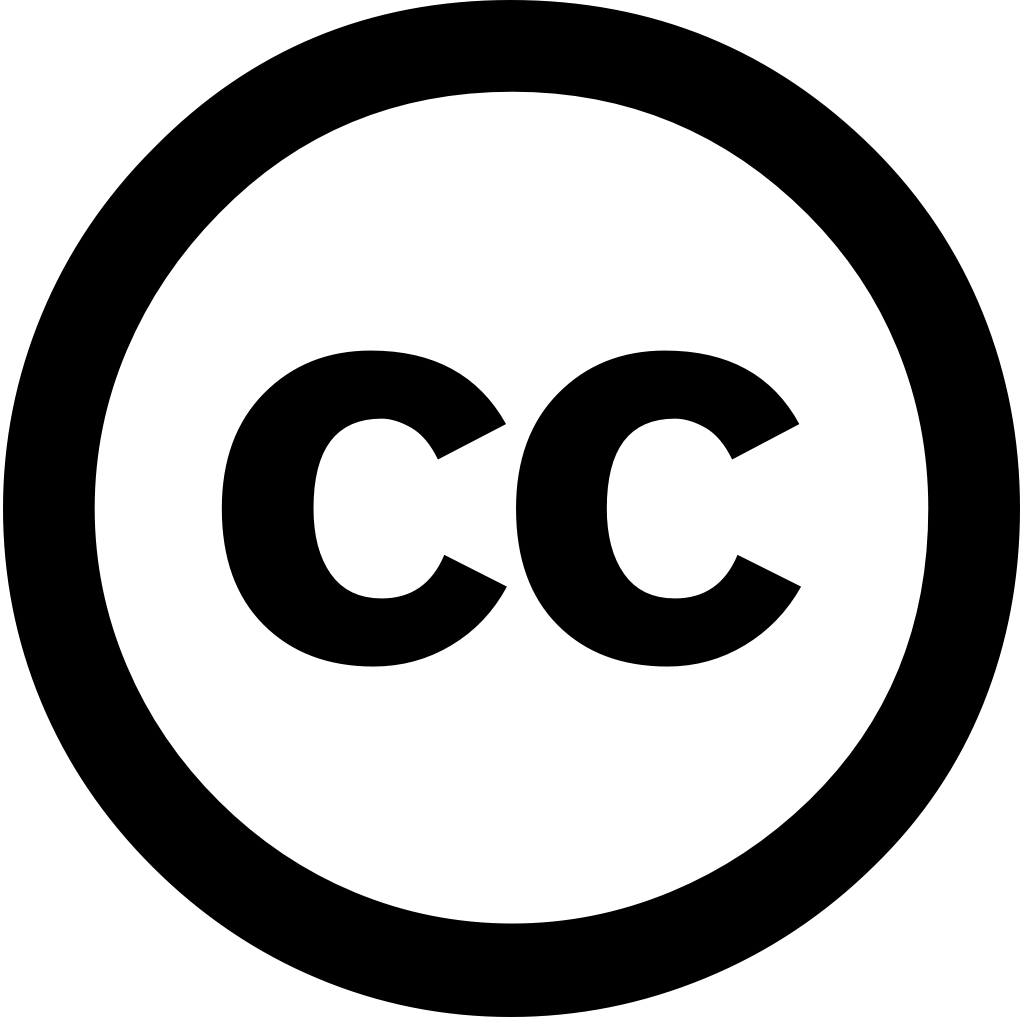
European Journal of Radiology, Journal Year: 2025, Volume and Issue: 186, P. 112049 - 112049
Published: March 14, 2025
Language: Английский
European Journal of Radiology, Journal Year: 2025, Volume and Issue: 186, P. 112049 - 112049
Published: March 14, 2025
Language: Английский
npj Digital Medicine, Journal Year: 2022, Volume and Issue: 5(1)
Published: Nov. 7, 2022
Abstract Machine learning is frequently being leveraged to tackle problems in the health sector including utilization for clinical decision-support. Its use has historically been focused on single modal data. Attempts improve prediction and mimic multimodal nature of expert decision-making met biomedical field machine by fusing disparate This review was conducted summarize current studies this identify topics ripe future research. We accordance with PRISMA extension Scoping Reviews characterize multi-modal data fusion health. Search strings were established used databases: PubMed, Google Scholar, IEEEXplore from 2011 2021. A final set 128 articles included analysis. The most common areas utilizing methods neurology oncology. Early merging strategy. Notably, there an improvement predictive performance when using fusion. Lacking papers clear deployment strategies, FDA-approval, analysis how approaches diverse sub-populations may biases healthcare disparities. These findings provide a summary as applied diagnosis/prognosis problems. Few compared outputs approach unimodal prediction. However, those that did achieved average increase 6.4% accuracy. Multi-modal learning, while more robust its estimations over methods, drawbacks scalability time-consuming information concatenation.
Language: Английский
Citations
216npj Digital Medicine, Journal Year: 2024, Volume and Issue: 7(1)
Published: March 14, 2024
Abstract Progress in sequencing technologies and clinical experiments has revolutionized immunotherapy on solid hematologic malignancies. However, the benefits of are limited to specific patient subsets, posing challenges for broader application. To improve its effectiveness, identifying biomarkers that can predict response is crucial. Machine learning (ML) play a pivotal role harnessing multi-omic cancer datasets unlocking new insights into immunotherapy. This review provides an overview cutting-edge ML models applied omics data analysis, including prediction immunotherapy-relevant tumor microenvironment identification. We elucidate how leverages diverse types identify significant biomarkers, enhance our understanding mechanisms, optimize decision-making process. Additionally, we discuss current limitations this rapidly evolving field. Finally, outline future directions aimed at overcoming these barriers improving efficiency research.
Language: Английский
Citations
23Environmental Toxicology, Journal Year: 2024, Volume and Issue: 39(10), P. 4479 - 4495
Published: March 15, 2024
The hypothesized link between low-density lipoprotein (LDL) and oncogenesis has garnered significant interest, yet its explicit impact on lung adenocarcinoma (LUAD) remains to be elucidated. This investigation aims demystify the function of LDL-related genes (LRGs) within LUAD, endeavoring shed light complex interplay LDL carcinogenesis.
Language: Английский
Citations
19BMC Medical Imaging, Journal Year: 2023, Volume and Issue: 23(1)
Published: Sept. 15, 2023
Abstract Background Vision transformer-based methods are advancing the field of medical artificial intelligence and cancer imaging, including lung applications. Recently, many researchers have developed vision AI for diagnosis prognosis. Objective This scoping review aims to identify recent developments on imaging It provides key insights into how transformers complemented performance deep learning cancer. Furthermore, also identifies datasets that contributed field. Methods In this review, we searched Pubmed, Scopus, IEEEXplore, Google Scholar online databases. The search terms included intervention (vision transformers) task (i.e., cancer, adenocarcinoma, etc.). Two reviewers independently screened title abstract select relevant studies performed data extraction. A third reviewer was consulted validate inclusion exclusion. Finally, narrative approach used synthesize data. Results Of 314 retrieved studies, 34 published from 2020 2022. most commonly addressed in these classification types, such as squamous cell carcinoma versus identifying benign malignant pulmonary nodules. Other applications survival prediction patients segmentation lungs. lacked clear strategies clinical transformation. SWIN transformer a popular choice researchers; however, other architectures were reported where combined with convolutional neural networks or UNet model. Researchers publicly available database consortium genome atlas. One study cluster 48 GPUs, while one, two, four GPUs. Conclusion can be concluded models increasingly popularity developing However, their computational complexity relevance important factors considered future research work. valuable healthcare advance state-of-the-art We provide an interactive dashboard lung-cancer.onrender.com/ .
Language: Английский
Citations
24Respiratory Research, Journal Year: 2025, Volume and Issue: 26(1)
Published: Jan. 18, 2025
Lipoid pneumonia (LP) is a rare disease caused by the accumulation of lipids and lipid-laden macrophages in alveoli inducing damage. LP difficult to differentiate from other similar diseases without pathological evidence, such as upper respiratory tract infection (URTI), pneumonia, cryptogenic organizing (COP), pulmonary alveolar proteinosis (PAP), lung mucinous adenocarcinoma edema. Given high misdiagnosis rate limited statistical clinical treatment data, there an urgent need for novel indicators LP. Superoxide dismutase type1 (SOD1) plays essential role macrophage polarization, promoting inflammation oxidative stress, but its association with remains unknown. The data 22 patients proven January 2008 June 2024 their prognostic information up were retrospectively gathered (ClinicalTrials.gov, NCT06430008). Additionally, on URTI, bacterial fungal COP, PAP, edema, was collected totaling 140 control subjects. Receiver operating characteristic curve, machine learning (ML), regression survival analyses performed analyze data. In multivariate analysis, sole independent risk factor level SOD1 (OR 0.922, 95% CI: 0.878 ~ 0.967, P < 0.001), while smoking status (β= -0.177, CI -18.645~-2.836, = 0.008), diabetes mellitus -0.191, -20.442~-3.592, 0.005), total sialic acid (TSA) -0.426, -0.915~ -0.433, 0.001) independently influenced SOD1. had highest importance score ML-based predictive models. advanced age may be associated higher mortality potential biomarker LP, status, comorbidities, TSA considered.
Language: Английский
Citations
1Cancer Letters, Journal Year: 2025, Volume and Issue: 612, P. 217494 - 217494
Published: Jan. 23, 2025
Language: Английский
Citations
1Journal of Medical Internet Research, Journal Year: 2024, Volume and Issue: 26, P. e48527 - e48527
Published: Jan. 22, 2024
Background Machine learning is a potentially effective method for predicting the response to platinum-based treatment ovarian cancer. However, predictive performance of various machine methods and variables still matter controversy debate. Objective This study aims systematically review relevant literature on value chemotherapy responses in patients with Methods Following PRISMA (Preferred Reporting Items Systematic Reviews Meta-Analyses) guidelines, we searched PubMed, Embase, Web Science, Cochrane databases studies models therapies cancer published before April 26, 2023. The Prediction Model Risk Bias Assessment tool was used evaluate risk bias included articles. Concordance index (C-index), sensitivity, specificity were prediction investigate platinum Results A total 1749 articles examined, 19 them involving 39 eligible this study. most commonly modeling logistic regression (16/39, 41%), Extreme Gradient Boosting (4/39, 10%), support vector 10%). training cohort reported C-index models, pooled 0.806; validation 12 0.831. Support performed well both cohorts, 0.942 0.879, respectively. sensitivity 0.890, 0.790 cohort. Conclusions can effectively predict how respond may provide reference development or updating subsequent scoring systems.
Language: Английский
Citations
7Annual Review of Biomedical Data Science, Journal Year: 2024, Volume and Issue: 7(1), P. 225 - 250
Published: May 20, 2024
The integration of multiomics data with detailed phenotypic insights from electronic health records marks a paradigm shift in biomedical research, offering unparalleled holistic views into and disease pathways. This review delineates the current landscape multimodal omics integration, emphasizing its transformative potential generating comprehensive understanding complex biological systems. We explore robust methodologies for ranging concatenation-based to transformation-based network-based strategies, designed harness intricate nuances diverse types. Our discussion extends incorporating large-scale population biobanks dissecting high-dimensional layers at single-cell level. underscores emerging role large language models artificial intelligence, anticipating their influence as near-future pivot approaches. Highlighting both achievements hurdles, we advocate concerted effort toward sophisticated models, fortifying foundation groundbreaking discoveries precision medicine.
Language: Английский
Citations
7Multimedia Tools and Applications, Journal Year: 2024, Volume and Issue: unknown
Published: Aug. 20, 2024
Language: Английский
Citations
7Genomics Proteomics & Bioinformatics, Journal Year: 2022, Volume and Issue: 20(5), P. 811 - 813
Published: Oct. 1, 2022
Citations
23