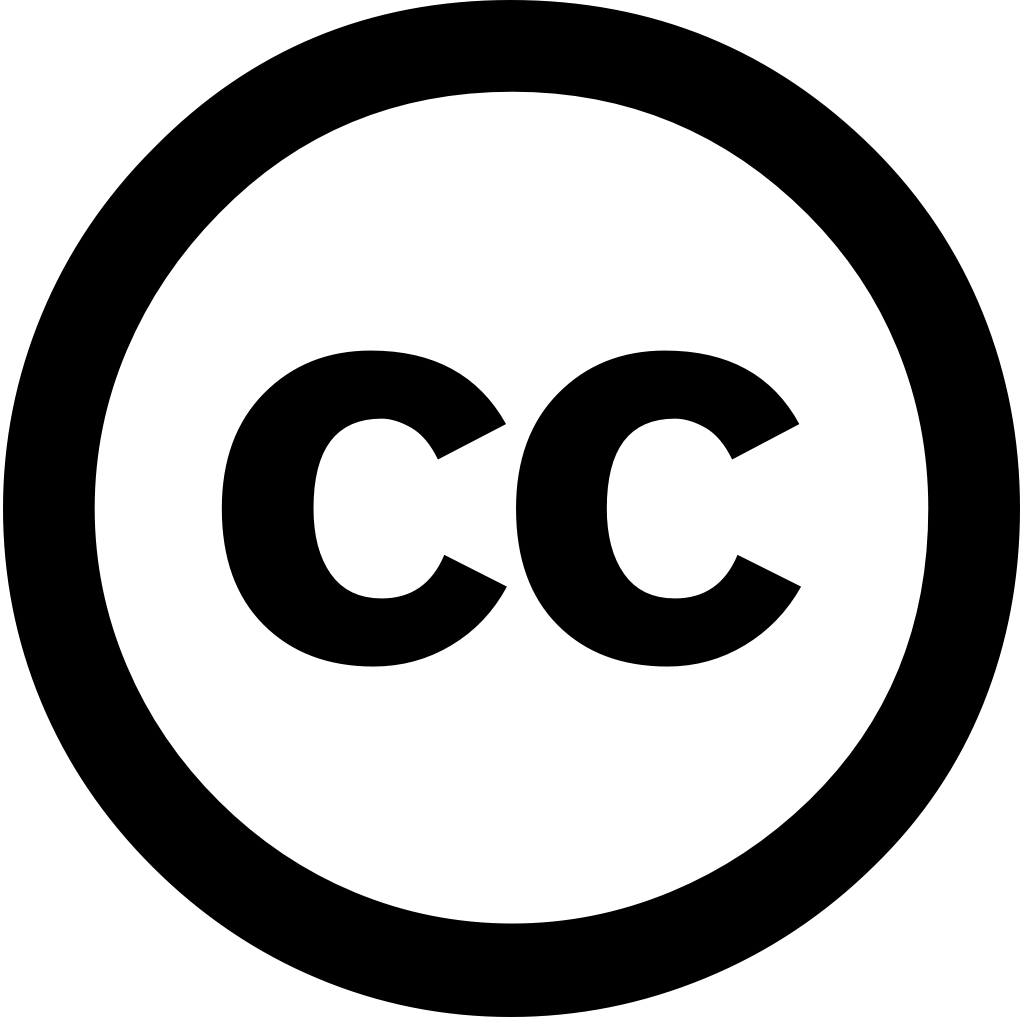
Science of Remote Sensing, Journal Year: 2025, Volume and Issue: unknown, P. 100231 - 100231
Published: May 1, 2025
Language: Английский
Science of Remote Sensing, Journal Year: 2025, Volume and Issue: unknown, P. 100231 - 100231
Published: May 1, 2025
Language: Английский
Information Fusion, Journal Year: 2024, Volume and Issue: 108, P. 102369 - 102369
Published: March 22, 2024
Wildfires have emerged as one of the most destructive natural disasters worldwide, causing catastrophic losses. These losses underscored urgent need to improve public knowledge and advance existing techniques in wildfire management. Recently, use Artificial Intelligence (AI) wildfires, propelled by integration Unmanned Aerial Vehicles (UAVs) deep learning models, has created an unprecedented momentum implement develop more effective Although survey papers explored learning-based approaches wildfire, drone disaster management, risk assessment, a comprehensive review emphasizing application AI-enabled UAV systems investigating role methods throughout overall workflow multi-stage including pre-fire (e.g., vision-based vegetation fuel measurement), active-fire fire growth modeling), post-fire tasks evacuation planning) is notably lacking. This synthesizes integrates state-of-the-science reviews research at nexus observations modeling, AI, UAVs - topics forefront advances elucidating AI performing monitoring actuation from pre-fire, through stage, To this aim, we provide extensive analysis remote sensing with particular focus on advancements, device specifications, sensor technologies relevant We also examine management approaches, monitoring, prevention strategies, well planning, damage operation strategies. Additionally, summarize wide range computer vision emphasis Machine Learning (ML), Reinforcement (RL), Deep (DL) algorithms for classification, segmentation, detection, tasks. Ultimately, underscore substantial advancement modeling cutting-edge UAV-based data, providing novel insights enhanced predictive capabilities understand dynamic behavior.
Language: Английский
Citations
43Ecological Indicators, Journal Year: 2024, Volume and Issue: 163, P. 112067 - 112067
Published: May 6, 2024
Deep learning techniques through semantic segmentation networks have been widely used for natural disaster analysis and response. The underlying base of these implementations relies on convolutional neural (CNNs) that can accurately precisely identify locate the respective areas interest within satellite imagery or other forms remote sensing data, thereby assisting in evaluation, rescue planning, restoration endeavours. Most CNN-based deep-learning models encounter challenges related to loss spatial information insufficient feature representation. This issue be attributed their suboptimal design layers capture multiscale-context failure include optimal during pooling procedures. In early CNNs, network encodes elementary representations, such as edges corners, whereas, progresses toward later layers, it more intricate characteristics, complicated geometric shapes. theory, is advantageous a extract features from several levels because generally yield improved results when both simple maps are employed together. study comprehensively reviews current developments deep methodologies segment images associated with disasters. Several popular models, SegNet U-Net, FCNs, FCDenseNet, PSPNet, HRNet, DeepLab, exhibited notable achievements various applications, including forest fire delineation, flood mapping, earthquake damage assessment. These demonstrate high level efficacy distinguishing between different land cover types, detecting infrastructure has compromised damaged, identifying regions fire-susceptible further dangers.
Language: Английский
Citations
17Remote Sensing of Environment, Journal Year: 2023, Volume and Issue: 295, P. 113723 - 113723
Published: July 18, 2023
Language: Английский
Citations
34Remote Sensing of Environment, Journal Year: 2023, Volume and Issue: 295, P. 113653 - 113653
Published: June 8, 2023
Over large areas, land cover classification has conventionally been undertaken using satellite time series. Typically temporal metric percentiles derived from single pixel location series have used to take advantage of spectral differences among classes over and minimize the impact missing observations. Deep convolutional neural networks (CNNs) demonstrated potential for date images. However, areas their application is complicated because they are sensitive observations may misclassify small spatially fragmented surface features due spatial patch-based implementation. This study demonstrates, first time, a one-dimensional (1D) CNN approach that uses percentile metrics does not these issues. all Conterminous United States (CONUS) considering two different 1D structures with 5 8 layers, respectively. CONUS 30 m classifications were available Landsat-5 -7 imagery seven-month growing season in 2011 3.3 million class labelled samples extracted contemporaneous National Land Cover Database (NLCD) 16 product. The CNNs and, conventional random forest model, trained 10%, 50% 90% samples, accuracies evaluated an independent 10% proportion. Temporal classified 5, 7 9 each five Landsat reflective wavelength bands eight band ratios. detailed 150 × km results demonstrate effective at scale locally. boundaries preserved axis dimension features, such as roads rivers, no stripes or anomalous patterns. 8-layer provided highest overall both 5-layer architectures higher than by 1.9% - 2.8% which > 83% meaningful increase. increased marginally number (86.21%, 86.40%, 86.43% percentiles, respectively) 1D-CNN. Class specific producer user quantified, lower developed land, crop pasture/hay classes, but systematic pattern respect used. Application model year ARD showed moderately decreased accuracy (80.79% percentiles) we illustrate likely intra-annual variations between years. These encouraging discussed recommended research deep learning percentiles.
Language: Английский
Citations
28The Science of The Total Environment, Journal Year: 2024, Volume and Issue: 923, P. 171367 - 171367
Published: March 1, 2024
Mangroves are an ecologically and economically valuable ecosystem that provides a range of ecological services, including habitat for diverse plant animal species, protection coastlines from erosion storms, carbon sequestration, improvement water quality. Despite their significant role, in many areas, Vietnam, large scale losses have occurred, although restoration efforts been underway. Understanding the loss efficacy requires high resolution temporal monitoring mangrove cover on scales. We produced time series 10-m-resolution maps using Multispectral Instrument Sentinel 2 satellites with this tool measured changes distribution Vietnamese Southern Coast (VSC). extracted annual ranging 2016 to 2023 deep learning model U-Net architecture based 17 spectral indices. Additionally, comparison misclassification by global products was conducted, indicating demonstrated superior performance when compared experiments multispectral bands Sentinel-2 time-series Sentinel-1 data, as shown highest performing The generated metrics, overall accuracy, precision, recall, F1-score were above 90 % entire years. Water indices investigated most important variables extraction. Our study revealed some misclassifications such World Cover Global Mangrove Watch highlighted significance our local analysis. While we did observe 34,778 ha (42.2 %) area region, 47,688 (57.8 new appeared, resulting net gain 12,910 (15.65 over eight-year period study. majority areas concentrated Ca Mau peninsulas within estuaries undergoing recovery programs natural processes. occurred regions where industrial development, wind farm projects, reclaimed land, shrimp pond expansion is occurring. theoretical framework well up-to-date data mapping change can be readily applied at other sites.
Language: Английский
Citations
14Science of Remote Sensing, Journal Year: 2025, Volume and Issue: unknown, P. 100213 - 100213
Published: Feb. 1, 2025
Language: Английский
Citations
1Journal of Imaging, Journal Year: 2022, Volume and Issue: 8(12), P. 317 - 317
Published: Nov. 24, 2022
In this paper, we propose an advanced scripting approach using Python and R for satellite image processing modelling terrain in Côte d'Ivoire, West Africa. Data include Landsat 9 OLI/TIRS C2 L1 the SRTM digital elevation model (DEM). The EarthPy library of 'raster' 'terra' packages are used as tools data processing. methodology includes computing vegetation indices to derive information on coverage modelling. Four were computed visualised R: Normalized Difference Vegetation Index (NDVI), Enhanced 2 (EVI2), Soil-Adjusted (SAVI) Atmospherically Resistant (ARVI2). SAVI index is demonstrated be more suitable better adjusted analysis, which beneficial agricultural monitoring d'Ivoire. analysis performed slope, aspect, hillshade relief with changed parameters sun azimuth angle. pattern d'Ivoire heterogeneous, reflects complexity structure. Therefore, aimed at relationship between regional topography environmental setting study area. upscaled mapping Yamoussoukro surroundings local topographic Kossou Lake. algorithms resampling, band composition, statistical map algebra calculation This demonstrates effective application programming
Language: Английский
Citations
28Measurement, Journal Year: 2023, Volume and Issue: 216, P. 112961 - 112961
Published: May 2, 2023
Language: Английский
Citations
14Remote Sensing, Journal Year: 2024, Volume and Issue: 16(5), P. 884 - 884
Published: March 2, 2024
Forest fires are caused by various climatic and anthropogenic factors. In Republic of Korea, forest occur frequently during spring when the humidity is low. During past decade, number fire incidents extent damaged area have increased. Satellite imagery can be applied to assess damage from these unpredictable fires. Despite increasing threat, there a lack comprehensive analysis effective strategies for addressing fires, particularly considering diverse topography Korea. Herein, we present an approach automated detection using Sentinel-2 images 14 areas affected in Korea 2019–2023. The performance deep learning (DL), machine learning, spectral index methods was analyzed, optimal model detecting derived. To evaluate independent models, two different burned exhibiting distinct characteristics were selected as test subjects. increase classification accuracy, tests conducted on combinations input channels DL. combination false-color RNG (B4, B8, B3) damage. Consequently, among DL HRNet achieved excellent results both regions with intersection over union scores 89.40 82.49, confirming that proposed method applicable Korean landscapes. Thus, suitable mitigation measures promptly designed based rapid areas.
Language: Английский
Citations
5ISPRS Journal of Photogrammetry and Remote Sensing, Journal Year: 2024, Volume and Issue: 218, P. 232 - 245
Published: Sept. 20, 2024
Language: Английский
Citations
5