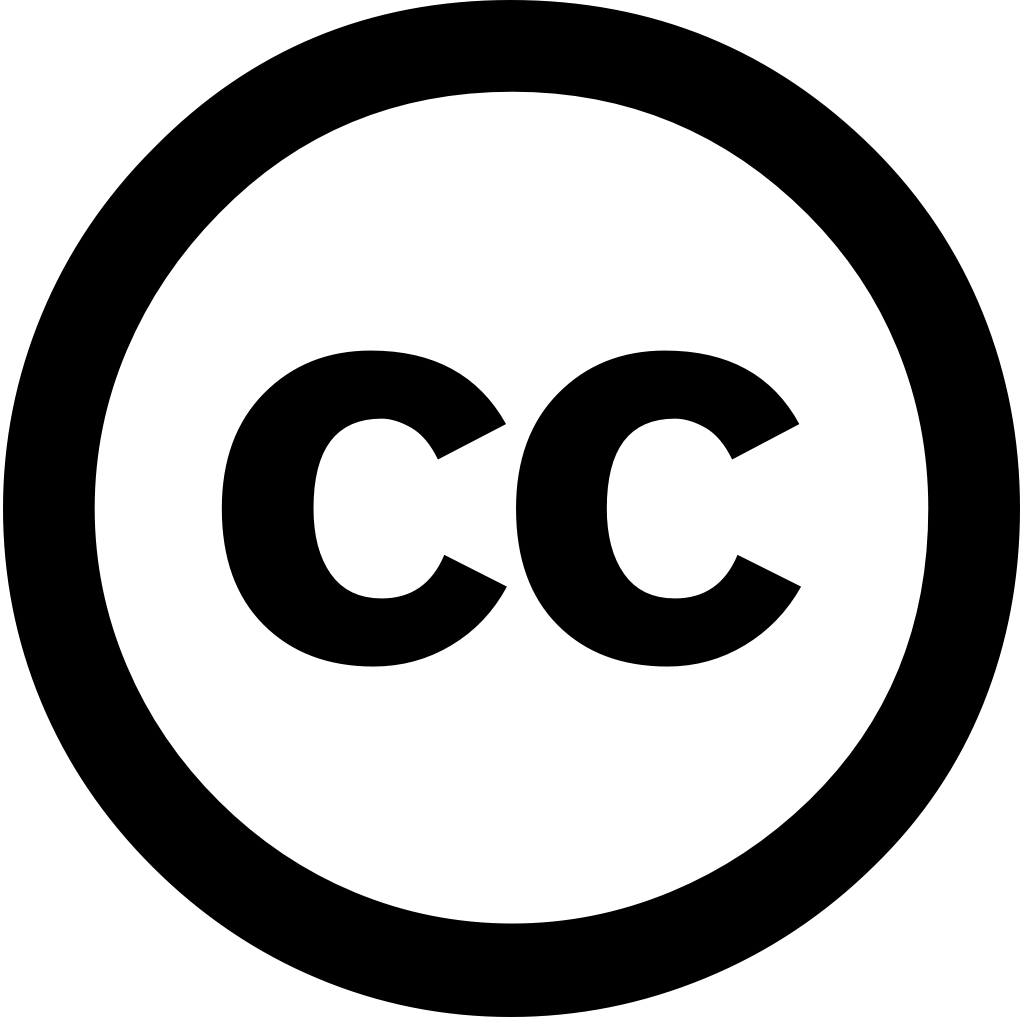
Scientific Reports, Journal Year: 2024, Volume and Issue: 14(1)
Published: Dec. 30, 2024
Machine vision was utilized in this study to accurately classify the low concentration slurry. Orthogonal experiment L
Language: Английский
Scientific Reports, Journal Year: 2024, Volume and Issue: 14(1)
Published: Dec. 30, 2024
Machine vision was utilized in this study to accurately classify the low concentration slurry. Orthogonal experiment L
Language: Английский
LatIA, Journal Year: 2024, Volume and Issue: 2, P. 74 - 74
Published: Sept. 29, 2024
Artificial intelligence (AI) holds significant potential to revolutionize healthcare by improving clinical practices and patient outcomes. This research explores the integration of AI in healthcare, focusing on methodologies such as machine learning, natural language processing, computer vision, which enable extraction valuable insights from complex medical imaging data. Through a comprehensive literature review, study highlights AI’s practical applications diagnostics, treatment planning, predicting Additionally, ethical issues, data privacy, legal frameworks are examined, emphasizing importance responsible usage healthcare. The findings demonstrate ability enhance diagnostic accuracy, streamline administrative tasks, optimize resource allocation, leading personalized treatments more efficient management. However, challenges remain, including quality, algorithm transparency, concerns, must be addressed ensure safe effective deployment. Continued research, collaboration between professionals experts, development robust regulatory essential for maximizing benefits while minimizing risks. underscores transformative stresses need multidisciplinary approach address complexities involved its widespread adoption
Language: Английский
Citations
18Journal of Imaging, Journal Year: 2025, Volume and Issue: 11(2), P. 59 - 59
Published: Feb. 15, 2025
Artificial intelligence (AI) transforms image data analysis across many biomedical fields, such as cell biology, radiology, pathology, cancer and immunology, with object detection, feature extraction, classification, segmentation applications. Advancements in deep learning (DL) research have been a critical factor advancing computer techniques for mining. A significant improvement the accuracy of detection algorithms has achieved result emergence open-source software innovative neural network architectures. Automated now enables extraction quantifiable cellular spatial features from microscope images cells tissues, providing insights into organization various diseases. This review aims to examine latest AI DL mining microscopy images, aid biologists who less background knowledge machine (ML), incorporate ML models focus images.
Language: Английский
Citations
3Cancers, Journal Year: 2024, Volume and Issue: 16(7), P. 1443 - 1443
Published: April 8, 2024
Cutaneous melanoma remains an increasing global public health burden, particularly in fair-skinned populations. Advancing technologies, artificial intelligence (AI), may provide additional tool for clinicians to help detect malignancies with a more accurate success rate. This systematic review aimed report the performance metrics of commercially available convolutional neural networks (CNNs) tasked detecting MM. A literature search was performed using CINAHL, Medline, Scopus, ScienceDirect and Web Science databases. total 16 articles reporting MM were included this review. The combined number melanomas detected 1160, non-melanoma lesions 33,010. market-approved technology clinician classifying highly heterogeneous, sensitivity ranging from 16.4 100.0%, specificity between 40.0 98.3% accuracy 44.0 92.0%. Less heterogeneity observed when worked unison AI, 83.3 83.7 87.3%, 86.4 86.9%. Instead focusing on AI versus melanoma, consistent has been obtained clinicians' work is supported by facilitating management decisions improving outcomes.
Language: Английский
Citations
6Intelligent Systems with Applications, Journal Year: 2025, Volume and Issue: unknown, P. 200529 - 200529
Published: April 1, 2025
Language: Английский
Citations
0i-manager’s Journal on Image Processing, Journal Year: 2025, Volume and Issue: 12(1), P. 63 - 63
Published: Jan. 1, 2025
Malaria continues to affect human lives extensively around the world, requiring urgent medical diagnostic procedures. This paper presents an improved version of U-Net deep learning method, which identifies malaria within microscopic blood smear images. The segmentation-based feature extraction offers superior performance when compared ordinary methods, thus leading better detection results. delivers precise location diseased areas, boosts accuracy, while CNN focuses on identification categories, and ANN faces difficulties identifying complex spatial patterns. experimental outcomes indicate that surpasses approaches by delivering higher values for sensitivity specificity. model provides exact results avoids mistakes shortening time. system is suited practical deployment besides offering optimal resources are limited. Data augmentation techniques improve overall generalization properties, makes resistant different datasets. A modern technological automated uses as its foundation.
Language: Английский
Citations
0Surgical Neurology International, Journal Year: 2025, Volume and Issue: 16, P. 174 - 174
Published: May 9, 2025
Background: This study aims to develop an artificial intelligence (AI) model using convolutional neural networks and transfer learning classify sellar barriers as strong, mixed, or weak based on magnetic resonance imaging (MRI). Accurate classification is essential for surgical planning in endoscopic endonasal approaches pituitary adenomas, variations the barrier can lead complications such cerebrospinal fluid leaks. Methods: The dataset consisted of 600 MRI images obtained from coronal sections evenly distributed among three classes. EfficientNetB0 architecture was employed, leveraging optimize performance despite small dataset. implemented trained Google Colab TensorFlow, with techniques dropout batch normalization improve generalization reduce overfitting. Performance metrics included accuracy, recall, F1-score. Results: AI achieved a accuracy 96.33%, individual class accuracies 98% strong barriers, 95% mixed 96% barriers. These results demonstrate model’s high capacity accurately identify their specific characteristics, ensuring reliable preoperative assessment. Conclusion: proposed significantly enhances contributing improving reducing complications. While “black box” nature poses challenges, integrating explainability modules expanding datasets further increase clinical trust applicability. underscores transformative potential neurosurgical practice, paving way precise diagnostics managing lesions.
Language: Английский
Citations
0Future Oncology, Journal Year: 2023, Volume and Issue: 19(40), P. 2651 - 2667
Published: Dec. 1, 2023
Aim: To develop a shiny app for doctors to investigate breast cancer treatments through new approach by incorporating unsupervised clustering and survival information. Materials & methods: Analysis is based on the Molecular Taxonomy of Breast Cancer International Consortium (METABRIC) dataset, which contains 1726 subjects 22 variables. Cox regression was used identify risk factors K-means clustering. Logrank tests C-statistics were compared across different cluster numbers Kaplan-Meier plots presented. Results conclusion: Our study fills an existing void introducing unique combination learning techniques information clinician side, demonstrating potential as valuable tool in uncovering hidden structures distinct profiles.
Language: Английский
Citations
9World Neurosurgery, Journal Year: 2024, Volume and Issue: 191, P. e403 - e410
Published: Sept. 2, 2024
The skull base is a complex region in neurosurgery, featuring numerous foramina. Accurate identification of these foramina imperative to avoid intraoperative complications and facilitate educational progress neurosurgical trainees. intricate landscape the often challenges both clinicians learners, necessitating innovative solutions. We aimed develop computer vision model that automates labeling from various image formats, enhancing surgical planning outcomes.
Language: Английский
Citations
3British Journal of Hospital Medicine, Journal Year: 2024, Volume and Issue: 85(7), P. 1 - 13
Published: July 30, 2024
Artificial intelligence has the potential to transform medical imaging. The effective integration of artificial into clinical practice requires a robust understanding its capabilities and limitations. This paper begins with an overview key use cases such as detection, classification, segmentation radiomics. It highlights foundational concepts in machine learning types strategies, well training evaluation process. We provide broad theoretical framework for assessing effectiveness imaging intelligence, including appraising internal validity generalisability studies, discuss barriers translation. Finally, we highlight future directions travel within field multi-modal data integration, federated explainability. By having awareness these issues, clinicians can make informed decisions about adopting imaging, improving patient care outcomes.
Language: Английский
Citations
2Innovative Surgical Sciences, Journal Year: 2024, Volume and Issue: unknown
Published: Aug. 19, 2024
Abstract Objectives Medical photography is ubiquitous and plays an increasingly important role in the fields of medicine surgery. Any assessment these photographs by computer vision algorithms requires first that area interest can accurately be delineated from background. We aimed to develop deep learning segmentation models for kidney liver organ donation where accurate automated has not yet been described. Methods Two novel (Detectron2 YoloV8) were developed using transfer compared against existing tools background removal (macBGRemoval, remBGisnet, remBGu2net). Anonymised photograph datasets comprised training/internal validation sets (821 400 images) external (203 208 images). Each image had two labels: whole clear view (parenchyma only). Intersection over Union (IoU) was primary outcome, as recommended metric assessing performance. Results In segmentation, Detectron2 YoloV8 outperformed other with internal IoU 0.93 0.94, 0.92 respectively. Other methods – macBGRemoval, remBGisnet remBGu2net scored lower, highest at 0.54 0.59. Similar results observed both showed 0.97 0.91, The a maximum 0.89 0.59 All tasks completed within 0.13–1.5 s per image. Conclusions Accurate, rapid context surgical possible open-source deep-learning software. These outperform could impact field surgery, enabling similar advancements seen areas medical vision.
Language: Английский
Citations
1