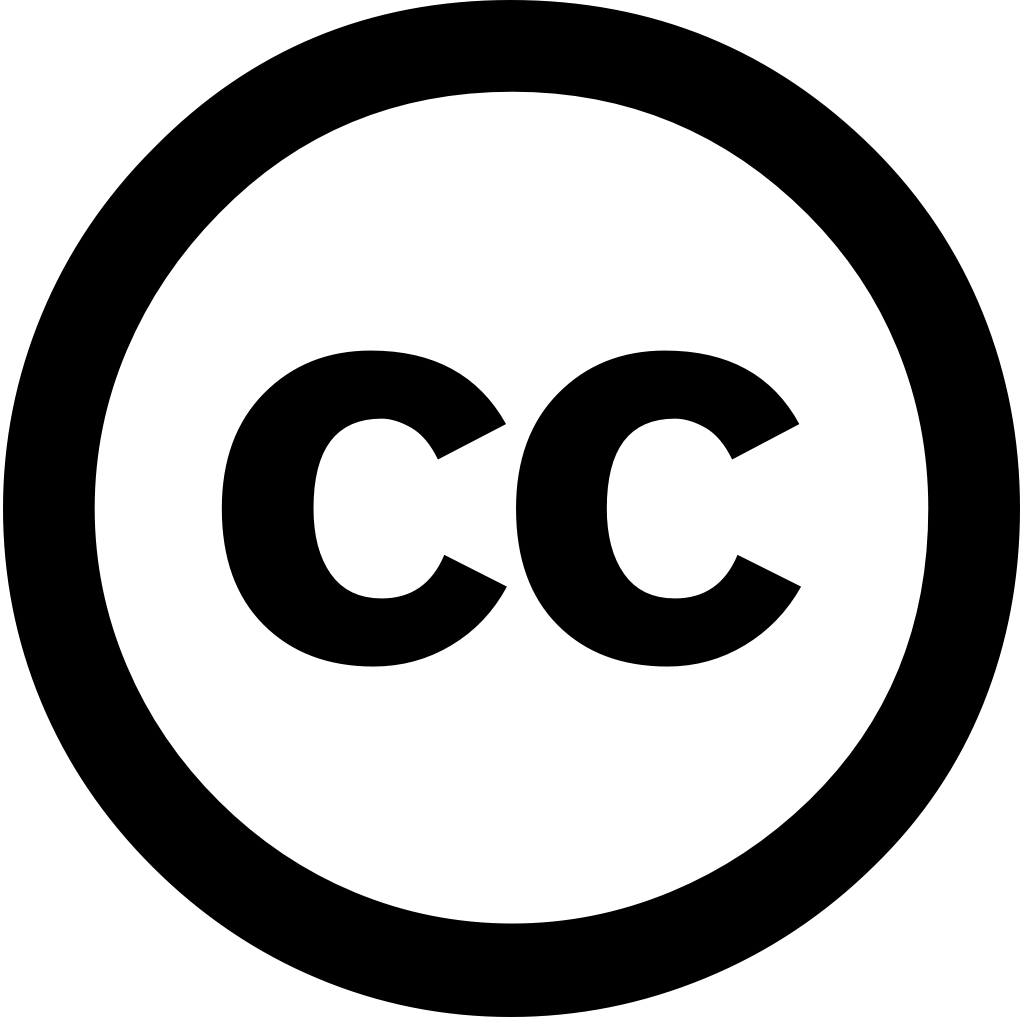
Soil and Water Research, Journal Year: 2022, Volume and Issue: 17(4), P. I - IV
Published: Nov. 2, 2022
Language: Английский
Soil and Water Research, Journal Year: 2022, Volume and Issue: 17(4), P. I - IV
Published: Nov. 2, 2022
Language: Английский
Journal of Soils and Sediments, Journal Year: 2025, Volume and Issue: unknown
Published: April 5, 2025
Language: Английский
Citations
1Environmental Science & Technology, Journal Year: 2024, Volume and Issue: 58(26), P. 11492 - 11503
Published: June 21, 2024
Soil organic carbon (SOC) plays a vital role in global cycling and sequestration, underpinning the need for comprehensive understanding of its distribution controls. This study explores importance various covariates on SOC spatial at both local (up to 1.25 km) continental (USA) scales using deep learning approach. Our findings highlight significant terrain attributes predicting concentration with terrain, contributing approximately one-third overall prediction scale. At scale, climate is only 1.2 times more important than distribution, whereas structural pattern 14 2 vegetation, respectively. We underscore that attributes, while being integral all scales, are stronger predictors scale explicit arrangement information. While this observational does not assess causal mechanisms, our analysis nonetheless presents nuanced perspective about which suggests disparate scales. The insights gained from have implications improved mapping, decision support tools, land management strategies, aiding development effective sequestration initiatives enhancing mitigation efforts.
Language: Английский
Citations
5Geoderma Regional, Journal Year: 2023, Volume and Issue: 34, P. e00658 - e00658
Published: June 8, 2023
Language: Английский
Citations
13The Science of The Total Environment, Journal Year: 2023, Volume and Issue: 883, P. 163677 - 163677
Published: April 25, 2023
The largest actively cycling terrestrial carbon pool, soil, has been disturbed during latest centuries by human actions through reduction of woody land cover. Soil organic (SOC) content can reliably be estimated in laboratory conditions, but more cost-efficient and mobile techniques are needed for large-scale monitoring SOC e.g. remote areas. We demonstrate the capability a hyperspectral camera operating visible-near infrared wavelength range practical estimation soil nitrogen content, to support efficient properties. 191 samples were collected Taita Taveta County, Kenya representing an altitudinal gradient comprising five typical use types: agroforestry, cropland, forest, shrubland sisal estate. imaged using Specim IQ under controlled their was determined with combustion analyzer. machine learning estimating N based on spectral images, studying also automatic selection informative wavelengths quantification prediction uncertainty. Five alternative methods all found perform well cross-validated R2 approximately 0.8 RMSE one percentage point, demonstrating feasibility proposed imaging setup computational pipeline.
Language: Английский
Citations
12Annals of Forest Science, Journal Year: 2024, Volume and Issue: 81(1)
Published: April 17, 2024
Abstract Key message The dataset includes data from forest soil surveys conducted in the period 2000–2020. It provides and site variables 8269 locations. Data are aggregated three basic layers: upper organic horizon (FH, 6875 locations), mineral layer 0–30 cm (M03, 8051 locations) deeper 30–80 (M38, 2260 locations). is available at https://doi.org/10.5281/zenodo.10608814 , access to metadata https://metadata-afs.nancy.inra.fr/geonetwork/srv/fre/catalog.search#/metadata/38f24573-3c0d-469a-a66a-7060ce082155 .
Language: Английский
Citations
4Soil and Water Research, Journal Year: 2025, Volume and Issue: unknown
Published: Jan. 3, 2025
Knowing the relationship between forest soil properties and their stand conditions is relevant for sustainable exploitation management of soils. This study examines influence environmental factors on within environments. We further assessed spatial variability these controlling factors. A harmonised database entire areas Czech Republic was considered; however, only 851 sampling points with complete data used out more than 8 thousand in database. The topsoil mineral layer 0–30 cm analysed. Principal component analysis to determine relationships nugget ratios semivariograms cross-variograms were evaluate dependence properties, Forest types reaction availability cations topsoils. Phosphorus influenced by aluminium cation exchange capacity. There are higher concentrations total phosphorus under broadleaved forest.
Language: Английский
Citations
0Environmental Monitoring and Assessment, Journal Year: 2025, Volume and Issue: 197(5)
Published: April 7, 2025
Language: Английский
Citations
0Soil and Water Research, Journal Year: 2024, Volume and Issue: 19(1), P. 32 - 49
Published: Jan. 16, 2024
Soil organic carbon (SOC) is an important soil characteristic as well a way how to mitigate climate change. Information on its content and spatial distribution thus crucial. Digital mapping (DSM) suitable evaluate of properties thanks ability obtain accurate information about soil. This research aims apply machine learning algorithms using various environmental covariates generate digital SOC maps for mineral topsoils in the Liberec Domažlice districts, located Czech Republic. The class, land cover, geology terrain extracted from elevation model remote sensing data were used modelling. was predicted based relationships with random forest (RF), cubist, quantile (QRF) models. Results RF showed that cover (vegetation) most variables prediction both districts. had better efficiency accuracy than cubist QRF predict greatest R2 value (0.63) observed district model. However, appropriate performance too.
Language: Английский
Citations
3Geoderma Regional, Journal Year: 2024, Volume and Issue: 38, P. e00855 - e00855
Published: Aug. 30, 2024
Language: Английский
Citations
3Smart Agricultural Technology, Journal Year: 2022, Volume and Issue: 3, P. 100106 - 100106
Published: Aug. 10, 2022
There are relatively few studies that explicitly evaluate the performance of machine learning algorithms (MLAs) such as decision trees while varying conditions like data splitting strategies and feature selection methods in digital soil mapping (DSM). Since several more powerful black-box models Random Forest (RF) exist, regular Classification Regression Tree (CART) least applied despite being intelligible than former. We demonstrate a simple yet relevant way to improve CART model for DSM still benefiting from its intelligibility, interpretability intuition potential. Soil organic carbon (SOC) levels across Czech Republic predicted at 30 m × resolution using selected covariates coupled with respective models. For this work, 440 topsoils (0–20 cm) were retrieved LUCAS database. Regarding distinct models, (Random, SPlit Conditional Latin Hypercube Sampling: cLHS) 7 varied. Meanwhile, overall results compared accuracy metrics including root mean square error (RMSE). One satisfactory SOC validation based on has (RMSE) 17.30 g/kg coefficient determination (R2) 0.52. The cLHS proves robust splitting. Feature Stepwise (SWR), Recursive Elimination (RFE) Genetic Algorithm (GA) considered computationally efficient identifying covariates. Generally, study demonstrates relevance effectiveness improving modelling via tree (CART).
Language: Английский
Citations
9