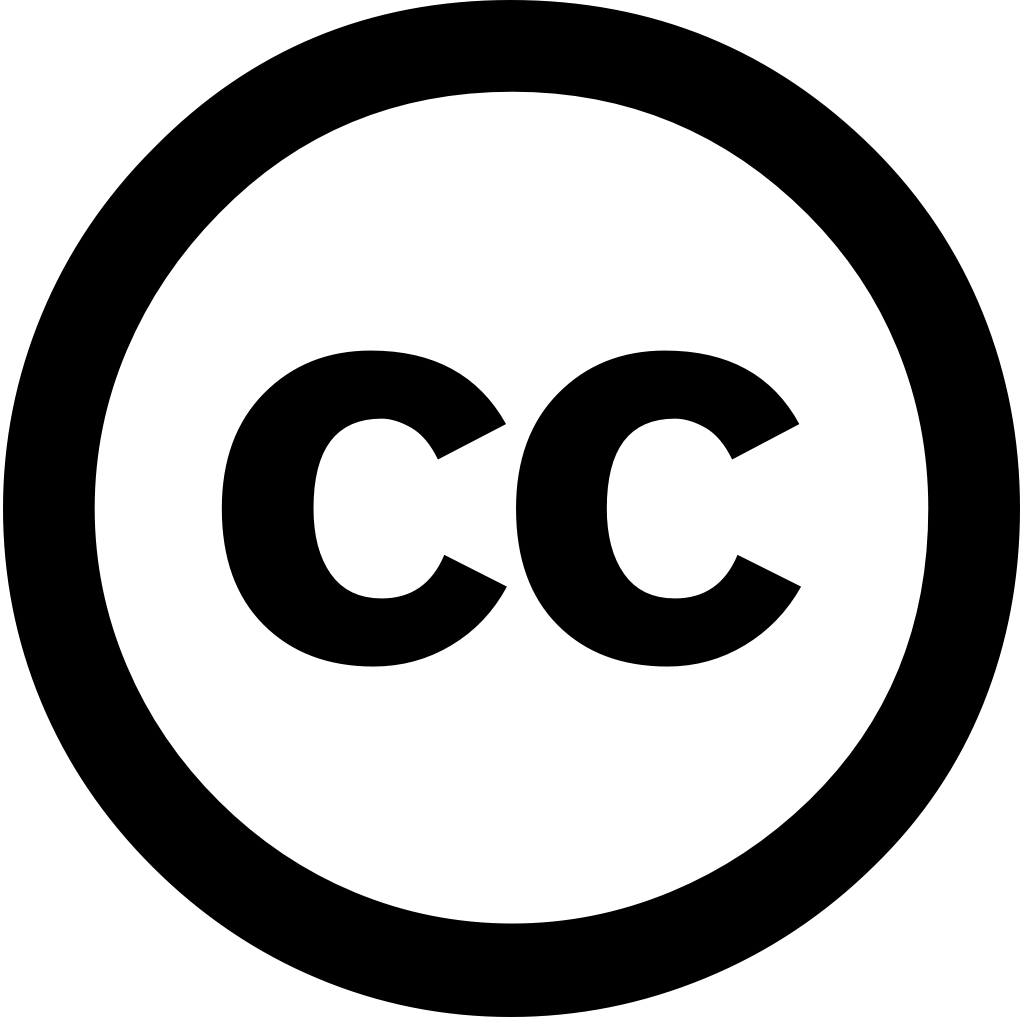
Journal of Rock Mechanics and Geotechnical Engineering, Journal Year: 2024, Volume and Issue: unknown
Published: Sept. 1, 2024
Language: Английский
Journal of Rock Mechanics and Geotechnical Engineering, Journal Year: 2024, Volume and Issue: unknown
Published: Sept. 1, 2024
Language: Английский
Natural Resources Research, Journal Year: 2024, Volume and Issue: 33(4), P. 1741 - 1762
Published: May 14, 2024
Language: Английский
Citations
22Geodata and AI., Journal Year: 2025, Volume and Issue: unknown, P. 100010 - 100010
Published: Feb. 1, 2025
Language: Английский
Citations
2Applied Sciences, Journal Year: 2023, Volume and Issue: 13(5), P. 3180 - 3180
Published: March 1, 2023
Rock image classification is a fundamental and crucial task in the creation of geological surveys. Traditional rock methods mainly rely on manual operation, resulting high costs unstable accuracy. While existing based deep learning models have overcome limitations traditional achieved intelligent classification, they still suffer from low accuracy due to suboptimal network structures. In this study, model EfficientNet triplet attention mechanism proposed achieve accurate end-to-end classification. The was built EfficientNet, which boasts an efficient structure thanks NAS technology compound scaling method, thus achieving for Additionally, introduced address shortcoming feature expression enable fully capture channel spatial information images, further improving During training, transfer employed by loading pre-trained parameters into model, accelerated convergence reduced training time. results show that with 92.6% set 93.2% Top-1 test set, outperforming other mainstream demonstrating strong robustness generalization ability.
Language: Английский
Citations
25Frontiers in Earth Science, Journal Year: 2023, Volume and Issue: 10
Published: Jan. 16, 2023
Rock image classification is a significant part of geological research. Compared with traditional methods, rock methods based on deep learning models have the great advantage in terms automatic features extraction. However, accuracies existing are unsatisfied due to weak feature extraction ability network model. In this study, residual neural (ResNet) model transfer method proposed establish corresponding for seven kinds images. ResNet34 introduces structure make it an excellent effect field classification, which extracts high-quality and avoids information loss. The abstracts from shallow features, better express texture case fewer To improve generalization model, total 3,82,536 images were generated training via slicing data augmentation. parameters trained Texture Library dataset contains 47 types reflect characteristics rocks used learning. This pre-trained weight loaded when dataset. Then fine-tuned problem. experimental results show that accuracy without reached 88.1%, while using achieved 99.1%. Aiming at engineering investigation, paper studies embedded deployment application network. transplanted platform. By designing system, off-line realized, provides new solution problem survey. can automatically classify manually extracting. These reduce influence subjective factors process more intelligent.
Language: Английский
Citations
23Computers & Geosciences, Journal Year: 2024, Volume and Issue: 188, P. 105600 - 105600
Published: April 28, 2024
Language: Английский
Citations
6Swiss Journal of Geosciences, Journal Year: 2024, Volume and Issue: 117(1)
Published: May 7, 2024
Abstract Deep subsurface exploration is important for mining, oil and gas industries, as well in the assessment of geological units disposal chemical or nuclear waste, viability geothermal energy systems. Typically, detailed examinations formations are performed on cuttings core materials extracted during drilling campaigns, geophysical borehole data, which provide information about petrophysical properties rocks. Depending volume rock samples analytical program, laboratory analysis diagnostics can be very time-consuming. This study investigates potential utilizing machine learning, specifically convolutional neural networks (CNN), to assess lithology mineral content solely from drill images, aiming support expedite exploration. The paper outlines a comprehensive methodology, encompassing data preprocessing, learning methods, transfer techniques. outcome reveals remarkable 96.7% accuracy classification segments into distinct formation classes. Furthermore, CNN model was trained evaluation using set multidimensional log (silicate, total clay, carbonate). When benchmarked against XRD measurements cores, both advanced network approach developed here equally good performance. work demonstrates that deep particularly extracting properties, including classification, thus offering road map enhancing performance quality image-based cores.
Language: Английский
Citations
4Computers & Geosciences, Journal Year: 2024, Volume and Issue: 191, P. 105664 - 105664
Published: June 21, 2024
Language: Английский
Citations
4Neurocomputing, Journal Year: 2023, Volume and Issue: 534, P. 55 - 66
Published: March 9, 2023
Language: Английский
Citations
10Scientific Reports, Journal Year: 2023, Volume and Issue: 13(1)
Published: Aug. 24, 2023
Recent advances in machine learning (ML) have transformed the landscape of energy exploration, including hydrocarbon, CO2 storage, and hydrogen. However, building competent ML models for reservoir characterization necessitates specific in-depth knowledge order to fine-tune achieve best predictions, limiting accessibility geosciences. To mitigate this issue, we implemented recently emerged automated (AutoML) approach perform an algorithm search conducting unconventional with a more optimized accessible workflow than traditional approaches. In study, over 1000 wells from Alberta's Athabasca Oil Sands were analyzed predict various key properties such as lithofacies, porosity, volume shale, bitumen mass percentage. Our proposed consists two stages AutoML (1) first stage focuses on predicting shale porosity by using conventional well log data, (2) second combines predicted outputs data lithofacies The findings show that out ten different tested (78% accuracy), (80.5%), percentage (67.3%), classification (98%), distributed random forest, gradient boosting models. When compared manually fine-tuned algorithms, AutoML-based algorithms provide notable improvement property higher weighted average f1-scores up 15-20% problem 5-10% adjusted-R2 score regression problems blind test dataset, it is achieved only after ~ 400 s training testing processes. addition, feature ranking extraction technique, there good agreement domain experts regarding most significant input parameters each prediction. Therefore, evidence has proven powerful performing advanced petrophysical analysis minimal time human intervention, allowing while maintaining model's explainability. Integration subject matter could advance artificial intelligence technology implementation optimizing data-driven
Language: Английский
Citations
10Neural Computing and Applications, Journal Year: 2025, Volume and Issue: unknown
Published: Jan. 9, 2025
Language: Английский
Citations
0