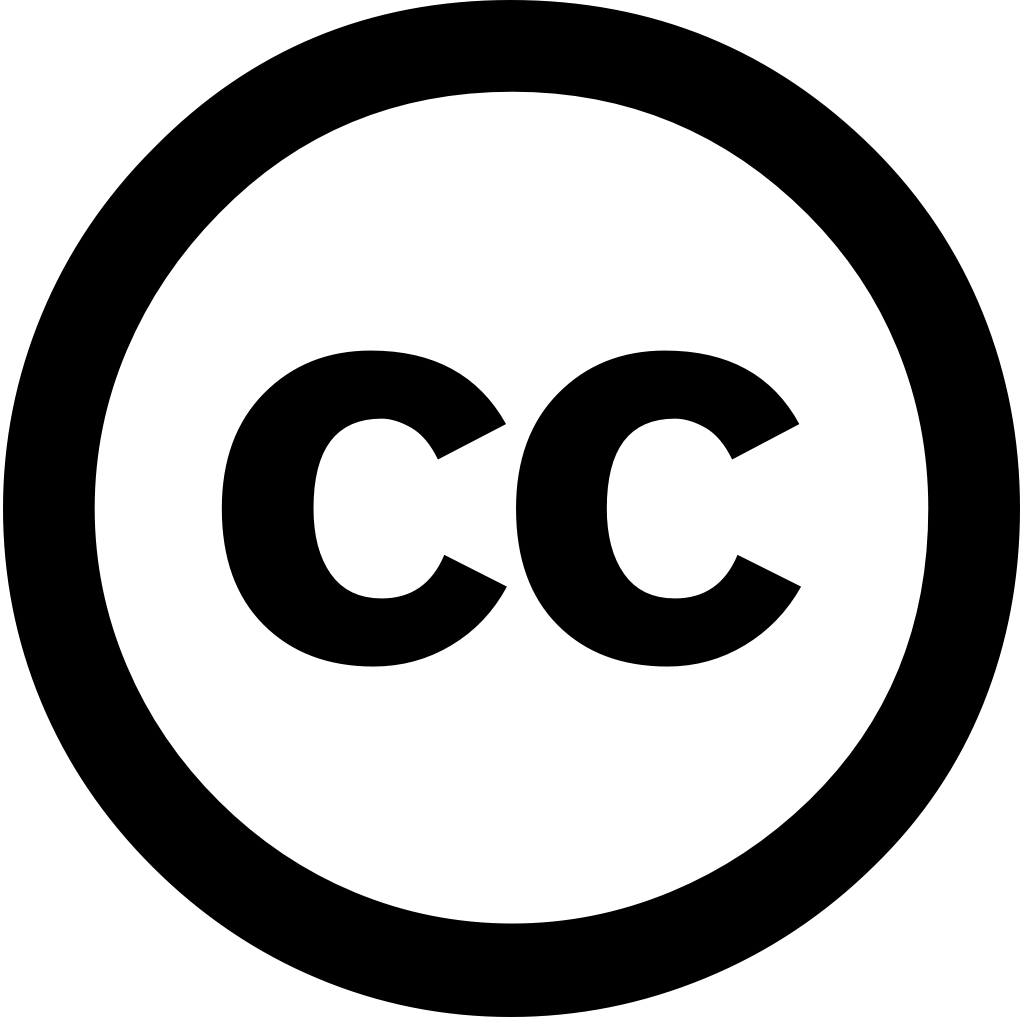
Computers in Biology and Medicine, Journal Year: 2025, Volume and Issue: 192, P. 110267 - 110267
Published: May 1, 2025
Language: Английский
Computers in Biology and Medicine, Journal Year: 2025, Volume and Issue: 192, P. 110267 - 110267
Published: May 1, 2025
Language: Английский
Proteomes, Journal Year: 2023, Volume and Issue: 11(4), P. 34 - 34
Published: Oct. 20, 2023
Multi-omics is a cutting-edge approach that combines data from different biomolecular levels, such as DNA, RNA, proteins, metabolites, and epigenetic marks, to obtain holistic view of how living systems work interact. has been used for various purposes in biomedical research, identifying new diseases, discovering drugs, personalizing treatments, optimizing therapies. This review summarizes the latest progress challenges multi-omics designing treatments human focusing on integrate analyze multiple proteome examples use multi-proteomics identify drug targets. We also discussed future directions opportunities developing innovative effective therapies by deciphering complexity.
Language: Английский
Citations
47Briefings in Bioinformatics, Journal Year: 2024, Volume and Issue: 25(3)
Published: March 27, 2024
Abstract Deep learning-based multi-omics data integration methods have the capability to reveal mechanisms of cancer development, discover biomarkers and identify pathogenic targets. However, current ignore potential correlations between samples in integrating data. In addition, providing accurate biological explanations still poses significant challenges due complexity deep learning models. Therefore, there is an urgent need for a method explore provide model interpretability. Herein, we propose novel interpretable (DeepKEGG) recurrence prediction biomarker discovery. DeepKEGG, hierarchical module designed local connections neuron nodes interpretability based on relationship genes/miRNAs pathways. pathway self-attention constructed correlation different generate feature representation enhancing performance model. Lastly, attribution-based importance calculation utilized related interpretation Experimental results demonstrate that DeepKEGG outperforms other state-of-the-art 5-fold cross validation. Furthermore, case studies also indicate serves as effective tool The code available at https://github.com/lanbiolab/DeepKEGG.
Language: Английский
Citations
18BMC Genomics, Journal Year: 2024, Volume and Issue: 25(1)
Published: Jan. 22, 2024
Abstract Background and objectives Comprehensive analysis of multi-omics data is crucial for accurately formulating effective treatment plans complex diseases. Supervised ensemble methods have gained popularity in recent years analysis. However, existing research based on supervised learning algorithms often fails to fully harness the information from unlabeled nodes overlooks latent features within among different omics, as well various associations features. Here, we present a novel integrative method MOSEGCN, Transformer multi-head self-attention mechanism Graph Convolutional Networks(GCN), with aim enhancing accuracy disease classification. MOSEGCN first employs Similarity Network Fusion (SNF) separately learn inherent correlations constructing comprehensive view Subsequently, it feeds learned into self-ensembling (SEGCN) built upon semi-supervised training testing, facilitating better utilization achieve precise classification subtypes. Results The experimental results show that outperforms several state-of-the-art approaches three types omics data: mRNA expression data, microRNA DNA methylation rates 83.0% Alzheimer's 86.7% breast cancer subtyping. Furthermore, exhibits strong generalizability GBM dataset, enabling identification important biomarkers related Conclusion explores significant relationship each omics' features, effectively leveraging labeled further enhance It also provides promising approach identifying reliable biomarkers, paving way personalized medicine.
Language: Английский
Citations
16British Journal of Cancer, Journal Year: 2024, Volume and Issue: 131(2), P. 205 - 211
Published: May 10, 2024
Abstract Multi-omics experiments at bulk or single-cell resolution facilitate the discovery of hypothesis-generating biomarkers for predicting response to therapy, as well aid in uncovering mechanistic insights into cellular and microenvironmental processes. Many methods data integration have been developed identification key elements that explain predict disease risk other biological outcomes. The heterogeneous graph representation multi-omics provides an advantage discerning patterns suitable predictive/exploratory analysis, thus permitting modeling complex relationships. Graph-based approaches—including neural networks—potentially offer a reliable methodological toolset can provide tangible alternative scientists clinicians seek ideas implementation strategies integrated analysis their omics sets biomedical research. workflows continue push limits technological envelope, this perspective focused literature review research articles which machine learning is utilized analyses, with several examples demonstrate effectiveness graph-based approaches.
Language: Английский
Citations
16Talanta, Journal Year: 2025, Volume and Issue: 287, P. 127693 - 127693
Published: Feb. 4, 2025
Language: Английский
Citations
4Frontiers in Genetics, Journal Year: 2023, Volume and Issue: 14
Published: July 20, 2023
Accurate diagnosis is the key to providing prompt and explicit treatment disease management. The recognized biological method for molecular of infectious pathogens polymerase chain reaction (PCR). Recently, deep learning approaches are playing a vital role in accurately identifying disease-related genes diagnosis, prognosis, treatment. models reduce time cost used by wet-lab experimental procedures. Consequently, sophisticated computational have been developed facilitate detection cancer, leading cause death globally, other complex diseases. In this review, we systematically evaluate recent trends multi-omics data analysis based on techniques their application prediction. We highlight current challenges field discuss how advances methods optimization overcoming them. Ultimately, review promotes development novel deep-learning methodologies integration, which essential
Language: Английский
Citations
37Seminars in Cancer Biology, Journal Year: 2023, Volume and Issue: 91, P. 35 - 49
Published: March 1, 2023
Language: Английский
Citations
29Computer Methods and Programs in Biomedicine, Journal Year: 2023, Volume and Issue: 231, P. 107377 - 107377
Published: Jan. 28, 2023
Language: Английский
Citations
27Genes, Journal Year: 2024, Volume and Issue: 15(4), P. 468 - 468
Published: April 8, 2024
In recent years, the FDA has approved numerous anti-cancer drugs that are mutation-based for clinical use. These have improved precision of treatment and reduced adverse effects side effects. Personalized therapy is a prominent hot topic current medicine also represents future direction development. With continuous advancements in gene sequencing high-throughput screening, research development strategies personalized developed rapidly. This review elaborates strategies, which include artificial intelligence, multi-omics analysis, chemical proteomics, computation-aided drug design. technologies rely on molecular classification diseases, global signaling network within organisms, new models all targets, significantly support medicine. Meanwhile, we summarize drugs, such as lorlatinib, osimertinib, other natural products, deliver therapeutic based genetic mutations. highlights potential challenges interpreting mutations combining while providing ideas pharmacogenomics cancer study.
Language: Английский
Citations
13BioData Mining, Journal Year: 2024, Volume and Issue: 17(1)
Published: Oct. 2, 2024
Language: Английский
Citations
13