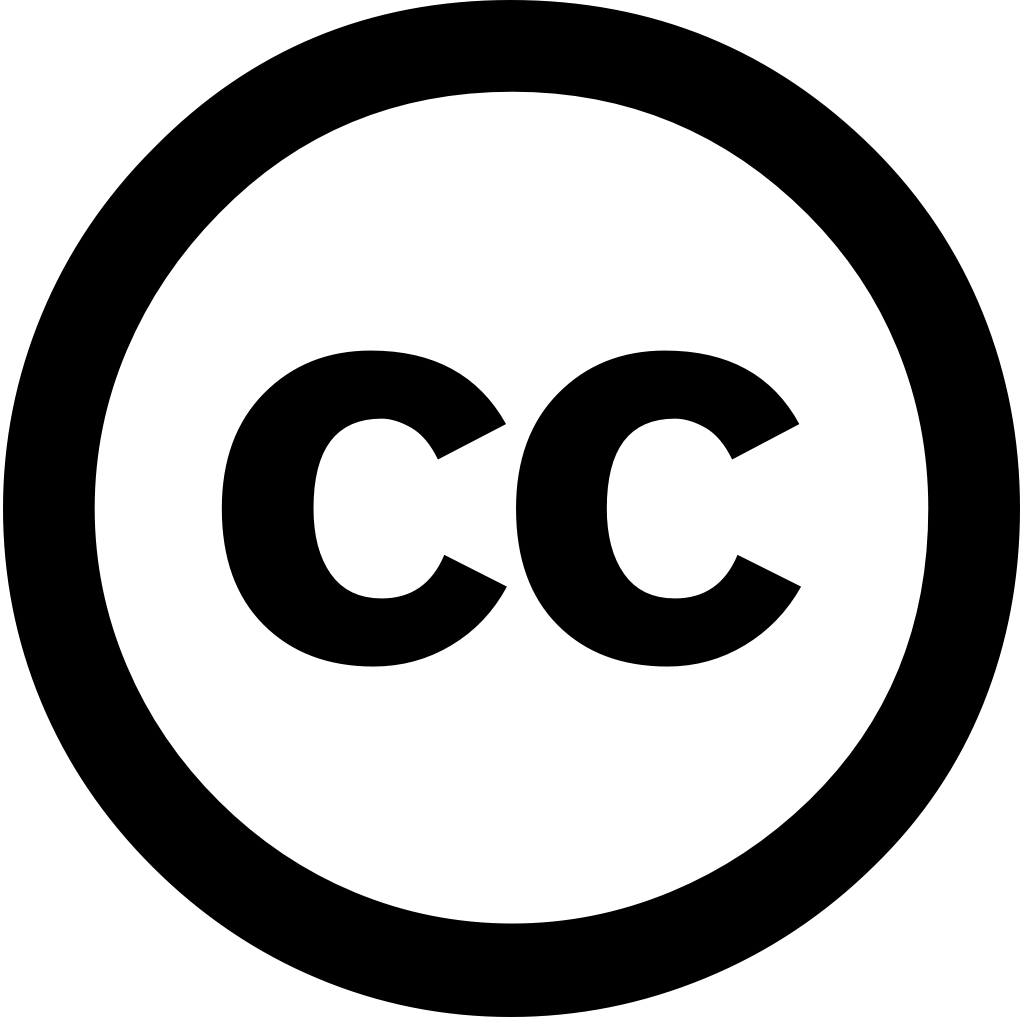
Applied Artificial Intelligence, Journal Year: 2025, Volume and Issue: 39(1)
Published: March 17, 2025
Language: Английский
Applied Artificial Intelligence, Journal Year: 2025, Volume and Issue: 39(1)
Published: March 17, 2025
Language: Английский
Computers and Electronics in Agriculture, Journal Year: 2024, Volume and Issue: 223, P. 109090 - 109090
Published: May 31, 2024
Language: Английский
Citations
56Expert Systems with Applications, Journal Year: 2024, Volume and Issue: 249, P. 123546 - 123546
Published: Feb. 21, 2024
Language: Английский
Citations
15Agriculture, Journal Year: 2024, Volume and Issue: 14(3), P. 353 - 353
Published: Feb. 22, 2024
Due to the challenges of pest detection in complex environments, this research introduces a lightweight network for tobacco identification leveraging enhancements YOLOv8 technology. Using large (YOLOv8l) as base, neck layer original is replaced with an asymptotic feature pyramid (AFPN) reduce model parameters. A SimAM attention mechanism, which does not require additional parameters, incorporated improve model’s ability extract features. The backbone network’s C2f VoV-GSCSP module computational requirements. Experiments show improved achieves high overall performance. Compared model, parameters and GFLOPs are reduced by 52.66% 19.9%, respectively, while [email protected] 1%, recall 2.7%, precision 2.4%. Further comparison popular models YOLOv5 medium (YOLOv5m), YOLOv6 (YOLOv6m), (YOLOv8m) shows has highest accuracy lightest detecting four common pests, optimal proposed facilitates precise, instantaneous recognition other crops, securing high-accuracy, comprehensive identification.
Language: Английский
Citations
13Computers and Electronics in Agriculture, Journal Year: 2024, Volume and Issue: 217, P. 108605 - 108605
Published: Jan. 20, 2024
Language: Английский
Citations
12Computers and Electronics in Agriculture, Journal Year: 2024, Volume and Issue: 218, P. 108700 - 108700
Published: Feb. 9, 2024
Language: Английский
Citations
10Food Research International, Journal Year: 2024, Volume and Issue: 194, P. 114929 - 114929
Published: Aug. 18, 2024
Language: Английский
Citations
9Agriculture, Journal Year: 2025, Volume and Issue: 15(2), P. 198 - 198
Published: Jan. 17, 2025
In unstructured tea garden environments, accurate recognition and pose estimation of bud leaves are critical for autonomous harvesting robots. Due to variations in imaging distance, exhibit diverse scale characteristics camera views, which significantly complicates the process. This study proposes a method using an RGB-D precise leaves. The approach first constructs leaves, followed by dynamic weight strategy achieve adaptive estimation. Quantitative experiments demonstrate that instance segmentation model achieves mAP@50 92.0% box detection 91.9% mask detection, improving 3.2% 3.4%, respectively, compared YOLOv8s-seg model. results indicate maximum angular error 7.76°, mean 3.41°, median 3.69°, absolute deviation 1.42°. corresponding distance errors 8.60 mm, 2.83 2.57 0.81 further confirming accuracy robustness proposed method. These can be applied environments non-destructive with bud-leave
Language: Английский
Citations
1Computers and Electronics in Agriculture, Journal Year: 2025, Volume and Issue: 231, P. 109944 - 109944
Published: Jan. 23, 2025
Language: Английский
Citations
1Industrial Crops and Products, Journal Year: 2025, Volume and Issue: 226, P. 120607 - 120607
Published: Feb. 1, 2025
Language: Английский
Citations
1Computers and Electronics in Agriculture, Journal Year: 2025, Volume and Issue: 233, P. 110061 - 110061
Published: Feb. 27, 2025
Language: Английский
Citations
1