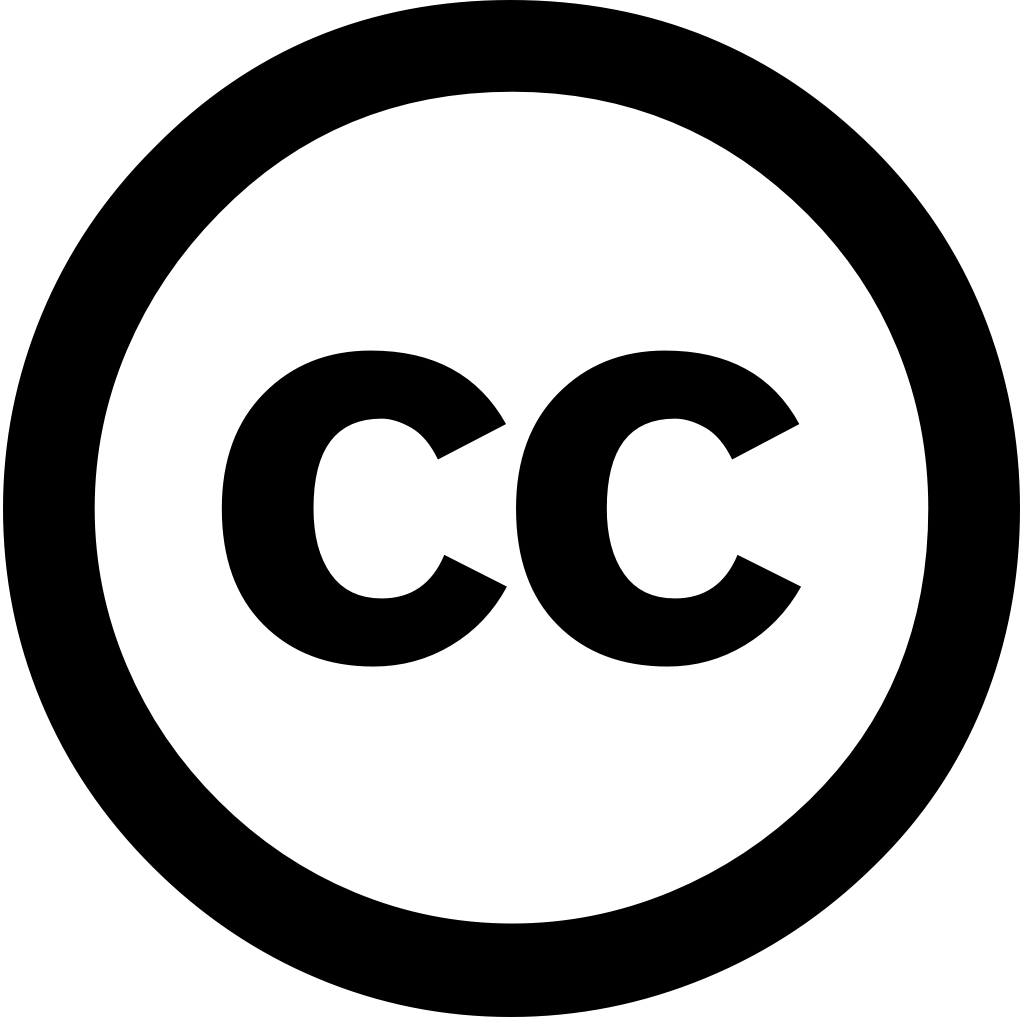
Scientific Reports, Journal Year: 2025, Volume and Issue: 15(1)
Published: May 9, 2025
Language: Английский
Scientific Reports, Journal Year: 2025, Volume and Issue: 15(1)
Published: May 9, 2025
Language: Английский
ACM Transactions on Intelligent Systems and Technology, Journal Year: 2023, Volume and Issue: 14(6), P. 1 - 50
Published: Sept. 11, 2023
Recent advancements in machine learning achieved by Deep Neural Networks (DNNs) have been significant. While demonstrating high accuracy, DNNs are associated with a huge number of parameters and computations, which leads to memory usage energy consumption. As result, deploying on devices constrained hardware resources poses significant challenges. To overcome this, various compression techniques widely employed optimize DNN accelerators. A promising approach is quantization, the full-precision values stored low bit-width precision. Quantization not only reduces requirements but also replaces high-cost operations low-cost ones. quantization offers flexibility efficiency design, making it adopted technique methods. Since has extensively utilized previous works, there need for an integrated report that provides understanding, analysis, comparison different approaches. Consequently, we present comprehensive survey concepts methods, focus image classification. We describe clustering-based methods explore use scale factor parameter approximating values. Moreover, thoroughly review training quantized DNN, including straight-through estimator regularization. explain replacement floating-point bitwise sensitivity layers quantization. Furthermore, highlight evaluation metrics important benchmarks classification task. accuracy state-of-the-art CIFAR-10 ImageNet. This article attempts make readers familiar basic advanced introduce works challenges future research this field.
Language: Английский
Citations
58Computers and Electronics in Agriculture, Journal Year: 2024, Volume and Issue: 223, P. 109090 - 109090
Published: May 31, 2024
Language: Английский
Citations
56Transactions of the Association for Computational Linguistics, Journal Year: 2024, Volume and Issue: 12, P. 1556 - 1577
Published: Jan. 1, 2024
Abstract Large Language Models (LLMs) have transformed natural language processing tasks successfully. Yet, their large size and high computational needs pose challenges for practical use, especially in resource-limited settings. Model compression has emerged as a key research area to address these challenges. This paper presents survey of model techniques LLMs. We cover methods like quantization, pruning, knowledge distillation, highlighting recent advancements. also discuss benchmarking strategies evaluation metrics crucial assessing compressed offers valuable insights researchers practitioners, aiming enhance efficiency real-world applicability LLMs while laying foundation future
Language: Английский
Citations
23Applied Intelligence, Journal Year: 2024, Volume and Issue: 54(22), P. 11804 - 11844
Published: Sept. 2, 2024
Abstract This paper critically examines model compression techniques within the machine learning (ML) domain, emphasizing their role in enhancing efficiency for deployment resource-constrained environments, such as mobile devices, edge computing, and Internet of Things (IoT) systems. By systematically exploring lightweight design architectures, it is provided a comprehensive understanding operational contexts effectiveness. The synthesis these strategies reveals dynamic interplay between performance computational demand, highlighting balance required optimal application. As models grow increasingly complex data-intensive, demand resources memory has surged accordingly. escalation presents significant challenges artificial intelligence (AI) systems real-world applications, particularly where hardware capabilities are limited. Therefore, not merely advantageous but essential ensuring that can be utilized across various domains, maintaining high without prohibitive resource requirements. Furthermore, this review underscores importance sustainable development. introduction hybrid methods, which combine multiple techniques, promises to deliver superior efficiency. Additionally, development intelligent frameworks capable selecting most appropriate strategy based on specific application needs crucial advancing field. practical examples engineering applications discussed demonstrate impact techniques. optimizing complexity efficiency, ensures advancements AI technology remain widely applicable. thus contributes academic discourse guides innovative solutions efficient responsible practices, paving way future Graphical abstract
Language: Английский
Citations
15ISPRS Journal of Photogrammetry and Remote Sensing, Journal Year: 2024, Volume and Issue: 209, P. 368 - 382
Published: Feb. 20, 2024
Remote sensing image scene classification (RSI-SC) is crucial for various high-level applications, including RSI retrieval, captioning, and object detection. Deep learning-based methods can accurately predict categories. However, these approaches often require numerous labeled samples training, limiting their practicality in real-world RS applications with scarce label resources. In contrast, few-shot remote (FS-RSI-SC) has garnered substantial research interest owing to its potential mitigate the need extensive training samples. recent years, there been a surge studies on FS-RSI-SC. This paper presents comprehensive overview of FS-RSI-SC research, categorizing existing into two groups. The first group comprises based data augmentation, transfer learning, metric meta-learning. Our analysis reveals that most fall meta-learning category, employing attention mechanisms, self-supervised learning (SSL), feature fusion techniques enhanced performance. Additionally, consistently outperform other this category. second centered around large-scale pre-training, which demonstrated remarkable competitiveness across tasks, special shown considerable expected attract more increasing popularity pre-training unimodal multimodal foundation models. Moreover, we proposed pipeline harnesses capabilities powerful large vision-language models (VLMs) as encoders, establishing new baselines commonly used datasets under standard experimental settings. empirical results validated effectiveness utilizing VLMs highlighted Through joint state-of-the-art our experiments VLMs, identified prevailing challenges outlined promising directions future research.
Language: Английский
Citations
11Internet of Things, Journal Year: 2025, Volume and Issue: unknown, P. 101553 - 101553
Published: March 1, 2025
Language: Английский
Citations
1Advanced Engineering Informatics, Journal Year: 2024, Volume and Issue: 62, P. 102786 - 102786
Published: Aug. 24, 2024
Language: Английский
Citations
8Signals and communication technology, Journal Year: 2024, Volume and Issue: unknown, P. 107 - 130
Published: Jan. 1, 2024
Language: Английский
Citations
7Sensors, Journal Year: 2024, Volume and Issue: 24(23), P. 7480 - 7480
Published: Nov. 23, 2024
This systematic review critically evaluates the current state and future potential of real-time, end-to-end smart, automated irrigation management systems, focusing on integrating Internet Things (IoTs) machine learning technologies for enhanced agricultural water use efficiency crop productivity. In this review, automation each component is examined in pipeline from data collection to application while analyzing its effectiveness, efficiency, integration with various precision agriculture technologies. It also investigates role interoperability, standardization, cybersecurity IoT-based solutions applications. Furthermore, existing gaps are identified proposed seamless across multiple sensor suites aiming achieve fully autonomous scalable management. The findings highlight transformative systems address global food challenges by optimizing maximizing yields.
Language: Английский
Citations
7Journal of Materials Informatics, Journal Year: 2024, Volume and Issue: 4(4)
Published: Dec. 31, 2024
Photocatalysis is a unique technology that harnesses solar energy through in-situ processes, operating without the need for external inputs. It integral to advancing environmental, energy, chemical, and carbon-neutral objectives, promoting dual goals of pollution control carbon reduction. However, conventional approach photocatalyst design faces challenges such as inefficiency, high costs, low success rates, highlighting integrating modern technologies seeking new paradigms. Here, we demonstrate comprehensive overview transformative strategies in design, combining computational materials science with deep learning technologies. The review covers fundamental principles followed by examination methods workflow deep-learning-assisted design. Deep approaches are extensively reviewed, focusing on discovery novel photocatalysts, microstructure property optimization, approaches, application exploration, mechanistic insights into photocatalysis. Finally, highlight synergy between multidimensional computation learning, while discussing future directions development. This offers summary offering not only enhance development photocatalytic but also expand practical applications photocatalysis various domains.
Language: Английский
Citations
7