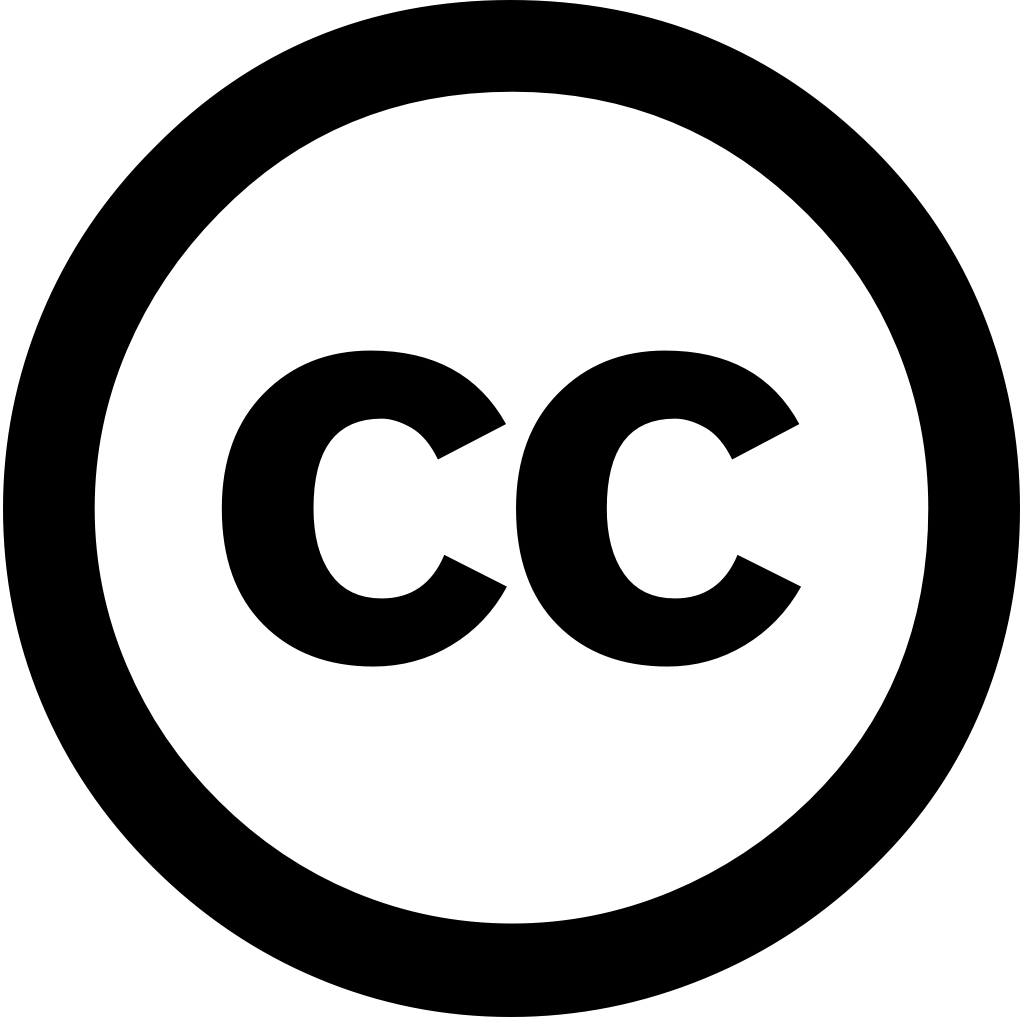
Current Opinion in Insect Science, Journal Year: 2024, Volume and Issue: 62, P. 101159 - 101159
Published: Jan. 9, 2024
Language: Английский
Current Opinion in Insect Science, Journal Year: 2024, Volume and Issue: 62, P. 101159 - 101159
Published: Jan. 9, 2024
Language: Английский
ICES Journal of Marine Science, Journal Year: 2023, Volume and Issue: 80(7), P. 1829 - 1853
Published: Aug. 3, 2023
Abstract Machine learning covers a large set of algorithms that can be trained to identify patterns in data. Thanks the increase amount data and computing power available, it has become pervasive across scientific disciplines. We first highlight why machine is needed marine ecology. Then we provide quick primer on techniques vocabulary. built database ∼1000 publications implement such analyse ecology For various types (images, optical spectra, acoustics, omics, geolocations, biogeochemical profiles, satellite imagery), present historical perspective applications proved influential, serve as templates for new work, or represent diversity approaches. Then, illustrate how used better understand ecological systems, by combining sources Through this coverage literature, demonstrate an proportion studies use learning, pervasiveness images source, dominance classification-type problems, shift towards deep all types. This overview meant guide researchers who wish apply methods their datasets.
Language: Английский
Citations
53Global Change Biology, Journal Year: 2021, Volume and Issue: 27(14), P. 3200 - 3217
Published: April 9, 2021
Climate-driven changes in the distribution of species are a pervasive and accelerating impact climate change, despite increasing research effort this rapidly emerging field, much remains unknown or poorly understood. We lack holistic understanding patterns processes at local, regional global scales, with detailed explorations range shifts southern hemisphere particularly under-represented. Australian waters encompass world's third largest marine jurisdiction, extending from tropical to sub-Antarctic zones, have warming rates twice average north two four times south. Here, we report results multi-taxon continent-wide review describing observed predicted redistribution around coastline, highlight critical gaps knowledge impeding our of, response to, these considerable changes. Since were first reported region 2003, 198 nine Phyla been documented shifting their distribution, 87.3% which poleward. However, there is little standardization methods metrics shifts, both hindered by baseline data. Our demonstrate importance historical data sets underwater visual surveys, also that approximately one-fifth studies incorporated citizen science. These findings emphasize important role public has had, can continue play, change. Most coastal fish sub-tropical temperate systems, while systems general explored. Moreover, most distributional only described poleward boundary, few considering warmer, equatorward limit. Through identifying limitations, highlights future opportunities for strategic improve representation climate-impact research.
Language: Английский
Citations
99Journal of Operational Oceanography, Journal Year: 2021, Volume and Issue: 14(sup1), P. 1 - 185
Published: Aug. 20, 2021
Chapter 1: CMEMS OSR5 1 1.1 IntroductionKarina von Schuckmann and Pierre-Yves Le Traon 1 1.2 Knowledge data for international Ocean governancePaula Kellett, Brittany E. Alexander Jo...
Language: Английский
Citations
97Frontiers in Marine Science, Journal Year: 2020, Volume and Issue: 7
Published: July 29, 2020
Spatial distributions of marine fauna are determined by complex interactions between environmental conditions and animal behaviors. As climate change leads to warmer, more acidic, less oxygenated oceans, species shifting away from their historical distribution ranges, these trends expected continue into the future. Correlative Species Distribution Models (SDMs) can be used project future habitat extent for species, with many different statistical methods available. However, it is vital assess how behave under novel before using models management advice, consider whether projections based on techniques biologically reasonable. In this study, we built SDMs adults larvae two ecologically important pelagic fishes in California Current System: Pacific sardine (Sardinops sagax) northern anchovy (Engraulis mordax). We five SDM methods, ranging simple (thermal niche model) (artificial neural networks). Our results show that some trained data collected 2003 2013 lost substantial predictive skill when applied observations recent years, ocean temperatures associated a heatwave were outside range measurements. This decrease was particularly apparent adult sardine, which showed non-stationary relationships catch locations sea surface temperature through time. While shifted markedly during heatwave, largely maintained spatiotemporal distributions. suggest correlative environment become unreliable anomalous conditions. Understanding underlying physiology therefore essential construction robust rapidly changing environments. Developing offer skillful predictions such as anchovy, migratory include separate sub-stocks, may challenging.
Language: Английский
Citations
73Global Change Biology, Journal Year: 2022, Volume and Issue: 28(22), P. 6586 - 6601
Published: Aug. 5, 2022
Projecting the future distributions of commercially and ecologically important species has become a critical approach for ecosystem managers to strategically anticipate change, but large uncertainties in projections limit climate adaptation planning. Although distribution are primarily used understand scope potential change-rather than accurately predict specific outcomes-it is nonetheless essential where why can give implausible results identify which processes contribute uncertainty. Here, we use series simulated distributions, an ensemble 252 models, three regional ocean projections, isolate influences uncertainty from earth system model spread ecological modeling. The simulations encompass marine with different functional traits preferences more broadly address resource manager fishery stakeholder needs, provide true state evaluate projections. We present our relative degree environmental extrapolation historical conditions, helps facilitate interpretation by modelers working diverse systems. found associated models exceed generated diverging (up 70% total 2100), that this result was consistent across traits. Species increased through time related extrapolated into novel conditions moderated how well captured underlying dynamics driving distributions. predictive power remained relatively high first 30 years alignment period stakeholders make strategic decisions based on information. By understanding sources uncertainty, they change at forecast horizons, recommendations projecting under global change.
Language: Английский
Citations
49Ecography, Journal Year: 2023, Volume and Issue: 2023(5)
Published: April 10, 2023
Species distribution models (SDMs) are widely used to relate species occurrence and density local environmental conditions, often include a spatially correlated variable account for spatial patterns in residuals. Ecologists have extended SDMs varying coefficients (SVCs), where the response given covariate varies smoothly over space time. However, SVCs see relatively little use perhaps because they remain less known relative other SDM techniques. We therefore review ecological contexts can improve interpretability descriptive power from SDMs, including responses regional indices that represent teleconnections; density‐dependent habitat selection; detectability; context‐dependent interactions with unmeasured covariates. then illustrate three additional examples detail using vector autoregressive spatio‐temporal (VAST) model. First, decadal trends model identifies arrowtooth flounder Atheresthes stomias Bering Sea 1982 2019. Second, trait‐based joint highlights role of body size temperature community assembly Gulf Alaska. Third, an age‐structured walleye pollock Gadus chalcogrammus contrasts cohorts broad distributions (1996 2009) those more constrained (2002 2015). conclude extend address wide variety be better understand range processes, e.g. dependence, population dynamics.
Language: Английский
Citations
27Ecological Informatics, Journal Year: 2024, Volume and Issue: 81, P. 102634 - 102634
Published: May 11, 2024
Large-scale modeling of environmental variables is an increasingly complex but necessary task. In this paper, we review the literature on using machine learning to cope with challenges associated spatial autocorrelation. Our focus was studies in which researchers predicted a supervised regression algorithm that accounted for autocorrelation any part pipeline from data exploration model validation. Methods included explicit covariates, splitting training–testing, calculations, and independent exploratory analysis. Authors most often analysis had no impact values. We concluded there seems be overall systematic approach how account models. selected studies, appropriate method depended specific characteristics study. Using covariates training-testing provided more insights into method's applicability. summarize these provide considerations selecting method.
Language: Английский
Citations
12International Journal of Geoheritage and Parks, Journal Year: 2025, Volume and Issue: unknown
Published: Feb. 1, 2025
Language: Английский
Citations
1Ecology and Evolution, Journal Year: 2020, Volume and Issue: 10(12), P. 5759 - 5784
Published: May 11, 2020
Abstract Species distribution models (SDMs) are important management tools for highly mobile marine species because they provide spatially and temporally explicit information on animal distribution. Two prevalent modeling frameworks used to develop SDMs generalized additive (GAMs) boosted regression trees (BRTs), but comparative studies have rarely been conducted; most rely presence‐only data; few explored how features such as characteristics affect model performance. Since the majority of BRTs predict habitat suitability, we first compared GAMs that presence/absence response variable. We then results from these suitability density (animals per km 2 ) built with a subset data here previously received extensive validation. both explanatory power (i.e., goodness fit) predictive performance novel dataset) taxonomically diverse suite cetacean using robust set systematic survey (1991–2014) within California Current Ecosystem. Both were successful at describing overall patterns throughout study area considered, when predicting data, exhibited substantially greater than BRTs, likely due different variables fitting algorithms. Our an improved understanding some strengths limitations developed two methods. These can be by modelers developing resource managers tasked spatial determine best technique their question interest.
Language: Английский
Citations
60PLoS ONE, Journal Year: 2020, Volume and Issue: 15(2), P. e0228065 - e0228065
Published: Feb. 5, 2020
Understanding the distribution of life's variety has driven naturalists and scientists for centuries, yet this been constrained both by available data models needed their analysis. Here we compiled over 67,000 marine terrestrial species used artificial neural networks to model richness with state variability climate, productivity, multiple other environmental variables. We find diversity is better predicted drivers than diversity, that can be a smaller set Ecological mechanisms such as geographic isolation structural complexity appear explain residuals also identify regions processes deserve further attention at global scale. Improving estimates relationships between patterns biodiversity, support them, should help in efforts mitigate impacts climate change provide guidance adapting life Anthropocene.
Language: Английский
Citations
58